DeLTA Seminar by Nicklas Werge
Speaker
Nicklas Werge, Ph.D. student at the Laboratory for Probability, Statistics and Modeling (LPSM), Sorbonne University.
Title
Non-Asymptotic Analysis of Stochastic Streaming Algorithms
Abstract
Motivated by the high-frequency data streams continuously generated, real-time learning is becoming increasingly important. These data streams should be processed sequentially with the property that the stream may change over time. In this streaming setting, we propose techniques for minimizing a convex objective through unbiased estimates of its gradients, commonly referred to as stochastic approximation problems. Our methods rely on stochastic approximation algorithms due to their computationally advantage as they only use the previous iterate as a parameter estimate. The reasoning includes iterate averaging that guarantees optimal statistical efficiency under classical conditions. Our non-asymptotic analysis shows accelerated convergence by selecting the learning rate according to the expected data streams. We show that the average estimate converges optimally and robustly to any data stream rate. In addition, noise reduction can be achieved by processing the data in a specific pattern, which is advantageous for large-scale machine learning. These theoretical results are illustrated for various data streams, showing the effectiveness of the proposed algorithms.
-------------------
Other upcoming DeLTA seminars:
29 November 2021 @ 10:00. Hippolyte Bourel.
-------------------
You can subscribe to DeLTA Seminar mailing list by sending an empty email to delta-seminar-join@list.ku.dk.
Information about DeLTA Seminars is available at the DeLTA Lab page: https://sites.google.com/diku.edu/delta.
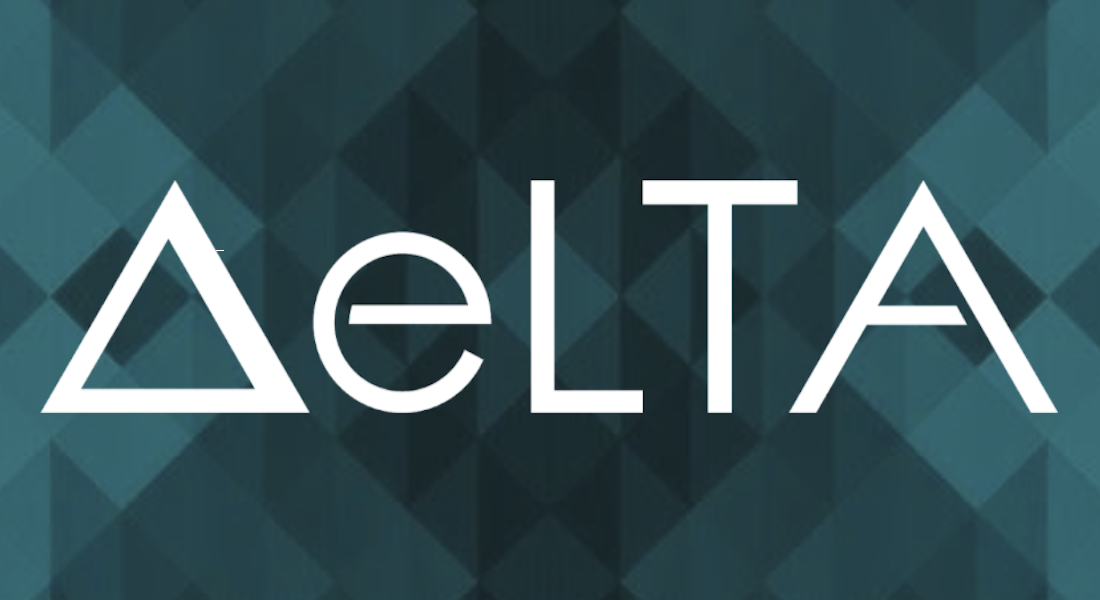
DeLTA is a research group affiliated with the Department of Computer Science at the University of Copenhagen studying diverse aspects of Machine Learning Theory and its applications, including, but not limited to Reinforcement Learning, Online Learning and Bandits, PAC-Bayesian analysis