DeLTA Seminar by Tomer Koren
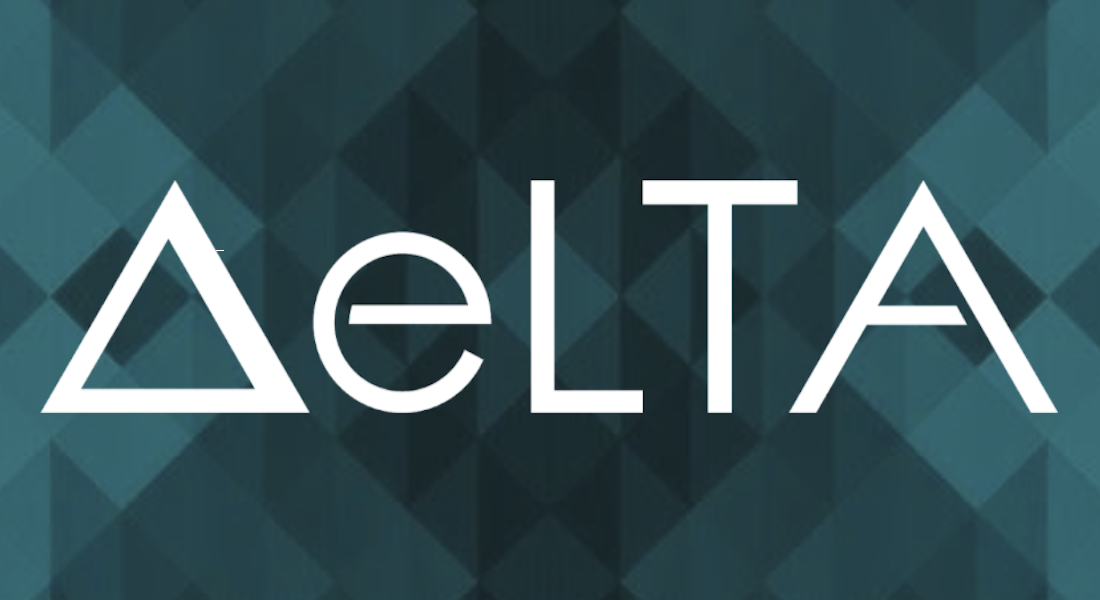
Title
Benign underfitting of Stochastic Gradient Descent, and some other peculiarities
Abstract
Why do gradient methods, such as Stochastic Gradient Descent (SGD), generalize well even when used to train predictors with far more parameters than training samples, and without any explicit form of capacity control (regularization)? In this talk, I will revisit this elusive question in the context of (stochastic) convex optimization and discuss some surprising theoretical results and phenomena that challenge the current conventional wisdom in machine learning.
Based on joint works with Idan Amir, Amit Attia, Roi Livni, Yishay Mansour, Uri Sherman and Matan Schliserman.
Speaker
Tomer Koren, Tel Aviv University.
Tomer has been an Assistant Professor in the Blavatnik School of Computer Science at Tel Aviv University since Fall 2019. Previously, he was a Senior Research Scientist at Google Brain, Mountain View. He received his PhD in 2017 from the Technion—Israel Institute of Technology.
His research interests are in machine learning and optimization.
-------------------
You can subscribe to the DeLTA Seminar mailing list by sending an empty email to delta-seminar-join@list.ku.dk.
Online calendar: https://calendar.google.com/calendar/embed?src=c_bm6u2c38ec3ti4lbfjd13c2aqg%40group.calendar.google.com&ctz=Europe%2FCopenhagen
DeLTA Lab page: https://sites.google.com/diku.edu/delta