DeLTA seminar by Andrea Tirinzoni: On Instance-optimal PAC Reinforcement Learning
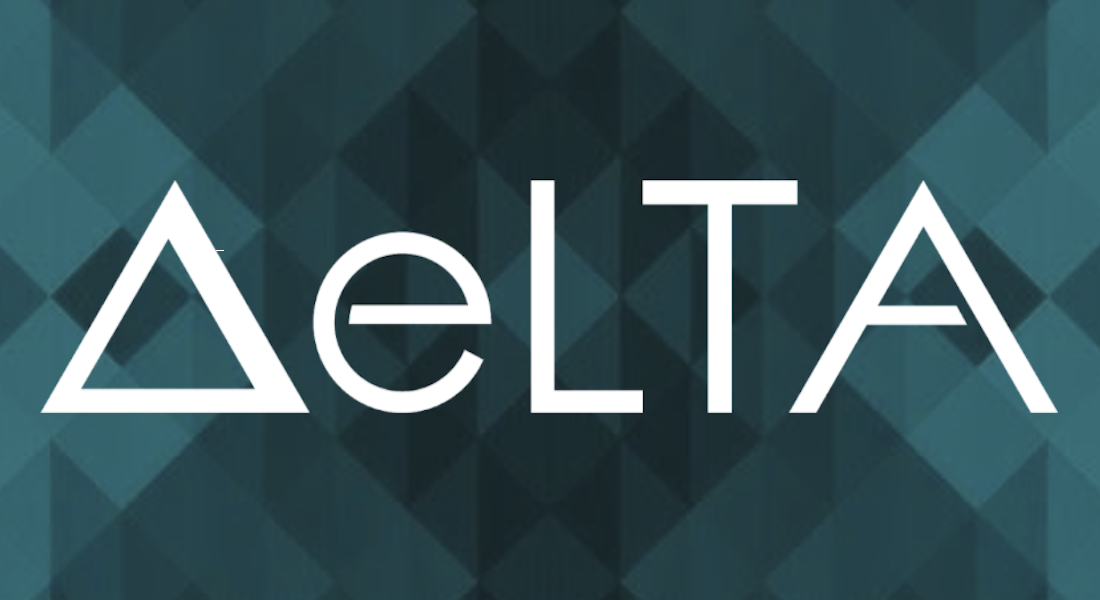
Speaker
Andrea Tirinzoni, postdoctoral researcher in the FAIR team at Meta (Paris).
Title
On Instance-optimal PAC Reinforcement Learning
Abstract
In probably approximately correct (PAC) reinforcement learning (RL), an agent is required to identify a near-optimal policy with high probability. While worst-case optimality has been attained for this problem, it is still unclear how an algorithm can optimally adapt to the complexity of the specific problem instance it faces. Toward addressing this limitation, in this talk, I will review some of our recent works on instance optimality in tabular episodic Markov decision processes (MDPs). First, for MDPs with deterministic transition dynamics, I will present an instance-dependent lower bound on the sample complexity of PAC RL and a computationally-efficient algorithm nearly matching it. While our lower bound is written as a linear program related to graph-theoretical concepts (minimum flows), our algorithm is very simple and does not require solving such an optimization problem explicitly. Second, for general MDPs with stochastic dynamics, I will show, through another instance-dependent lower bound, that the PEDEL algorithm by Wagenmaker and Jamieson (2022) is near instance-optimal. Considering the intractability of PEDEL, I will formulate an open question regarding the possibility of achieving our lower bound using a computationally-efficient algorithm.
_____________________________
You can subscribe to the DeLTA Seminar mailing list by sending an empty email to delta-seminar-join@list.ku.dk.
Online calendar
DeLTA Lab page
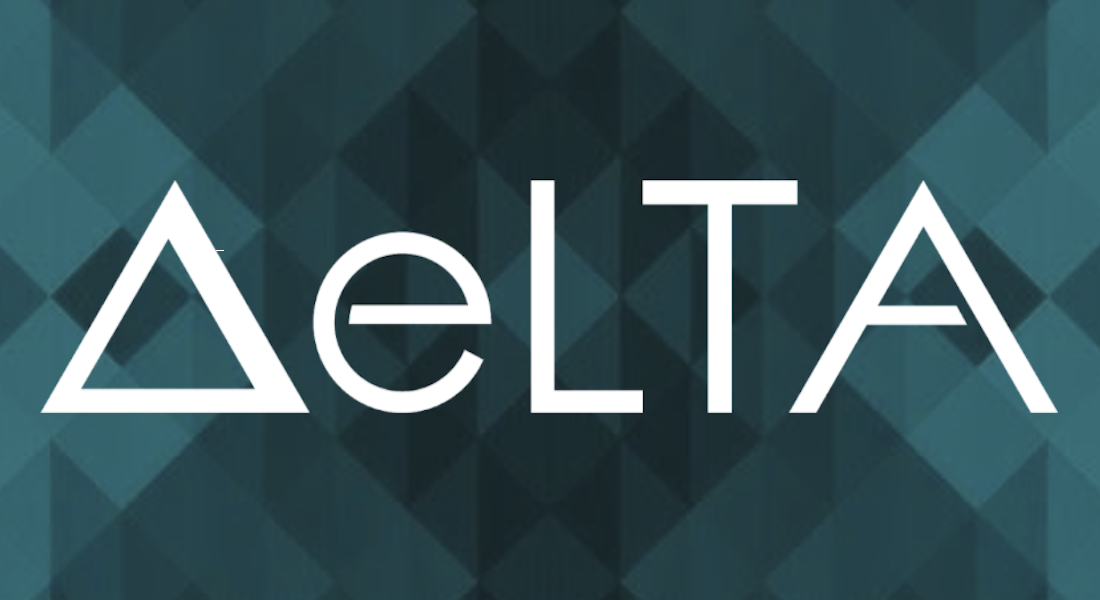
DeLTA is a research group affiliated with the Department of Computer Science at the University of Copenhagen studying diverse aspects of Machine Learning Theory and its applications, including, but not limited to Reinforcement Learning, Online Learning and Bandits, PAC-Bayesian analysis