DeLTA seminar by Daniil Dmitrievs: Lower Bounds for Online Private Learning
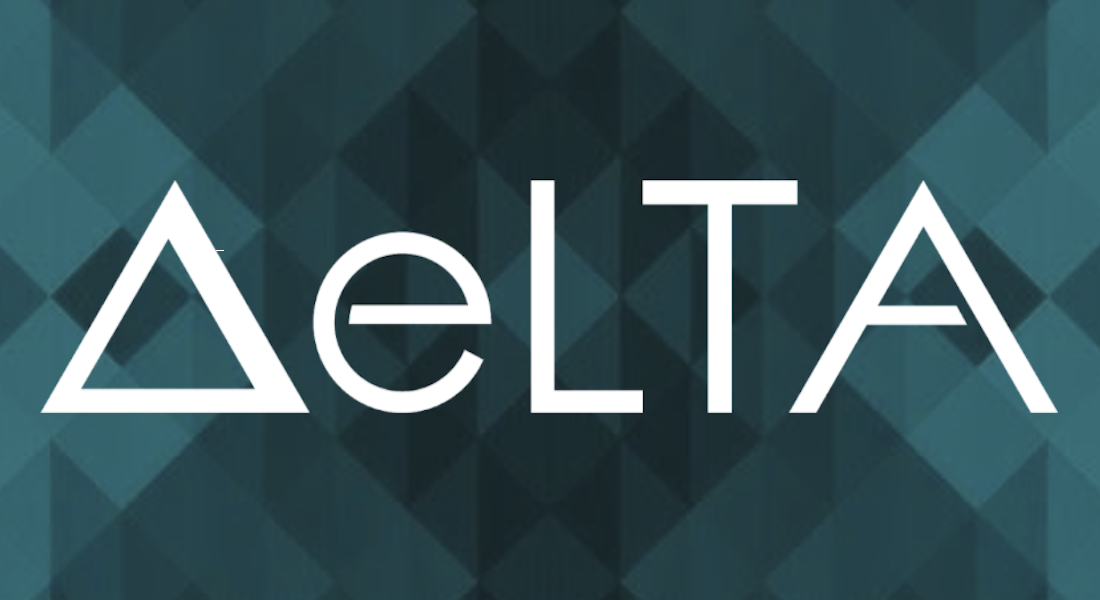
Speaker
Daniil Dmitriev, ETH AI Center Doctoral Fellow at ETH Zürich.
Title
Lower Bounds for Online Private Learning
Abstract
Online learning is a widely studied problem in learning theory. In the mistake bound model, the goal is to learn a target function from a sequence of arbitrary (non-stochastic) points, by making as few mistakes as possible. Differential privacy is a property of the algorithm that restricts its output to not strongly depend on any particular input. Recent work showed that function classes that are learnable in the offline model with differential privacy constraints are exactly those that are online learnable in the mistake bound model. In this work, we study which function classes are online learnable (in the mistake bound model) under differential privacy. Existing results provide an upper bound for the number of mistakes a private algorithm will commit in this model. This upper bound depends logarithmically on the number of steps, in contrast to classical (non-private) bounds for online learning. We prove that there exists a function class, where this dependency is required, i.e., it cannot be privately learned in the mistake bound model with small number of mistakes. Furthermore, we argue that this result holds much more broadly, for almost any function class. This is joint work with Amartya Sanyal and Kristóf Szabó.
_____________________________
You can subscribe to the DeLTA Seminar mailing list by sending an empty email to delta-seminar-join@list.ku.dk.
Online calendar
DeLTA Lab page
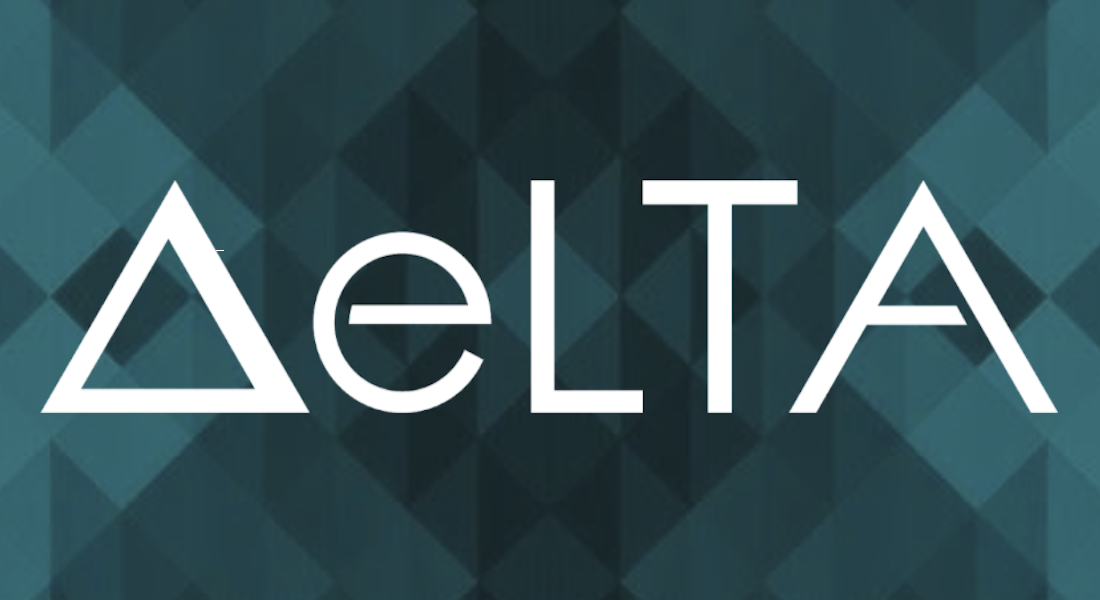
DeLTA is a research group affiliated with the Department of Computer Science at the University of Copenhagen studying diverse aspects of Machine Learning Theory and its applications, including, but not limited to Reinforcement Learning, Online Learning and Bandits, PAC-Bayesian analysis