DeLTA seminar by Nikita Zhivotovskiy: Improving Risk Bounds with Unbounded Losses via Data-Dependent Priors
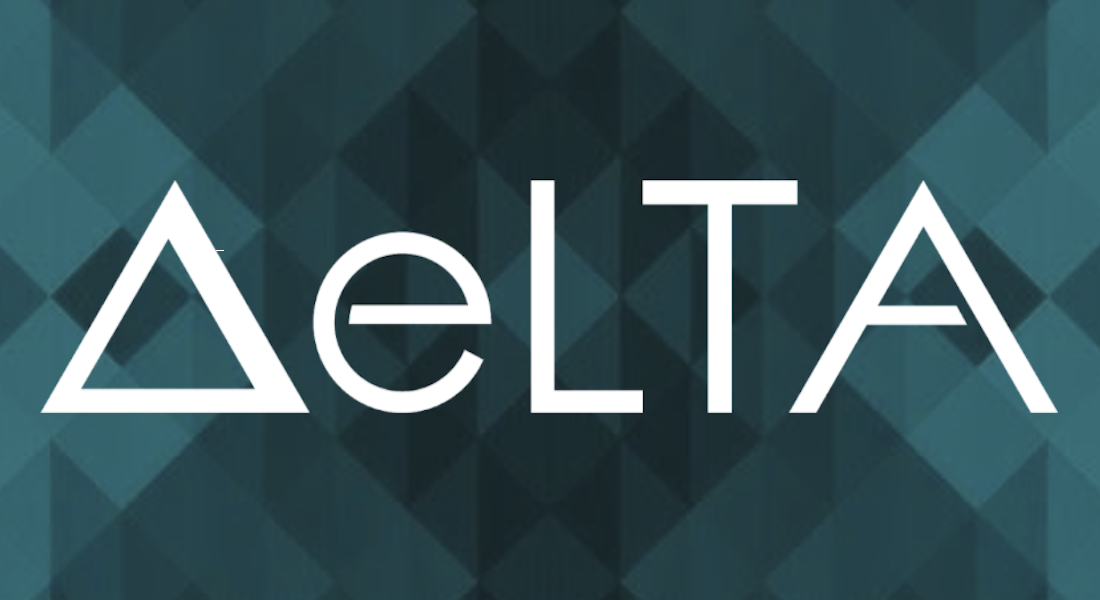
Speaker
Nikita Zhivotovskiy, UC Berkley
Title
Improving Risk Bounds with Unbounded Losses via Data-Dependent Priors
Abstract
In this talk, we revisit sequential linear regression, classification, and logistic regression, using scenarios where design vectors are known in advance but unordered. We discuss how this allows us to convert bounds into statistical ones with random design without additional assumptions. Using the exponential weights algorithm and data-dependent priors, we manage unbounded norms of optimal solutions. We show our classification regret bounds depend only on dimension and rounds, not on design vectors or norms. We argue these bounds are unique to this setting and unattainable in worst-case setups, offering polynomial-time algorithms involving log-concave sampling.
_____________________________
You can subscribe to the DeLTA Seminar mailing list by sending an empty email to delta-seminar-join@list.ku.dk.
Online calendar
DeLTA Lab page
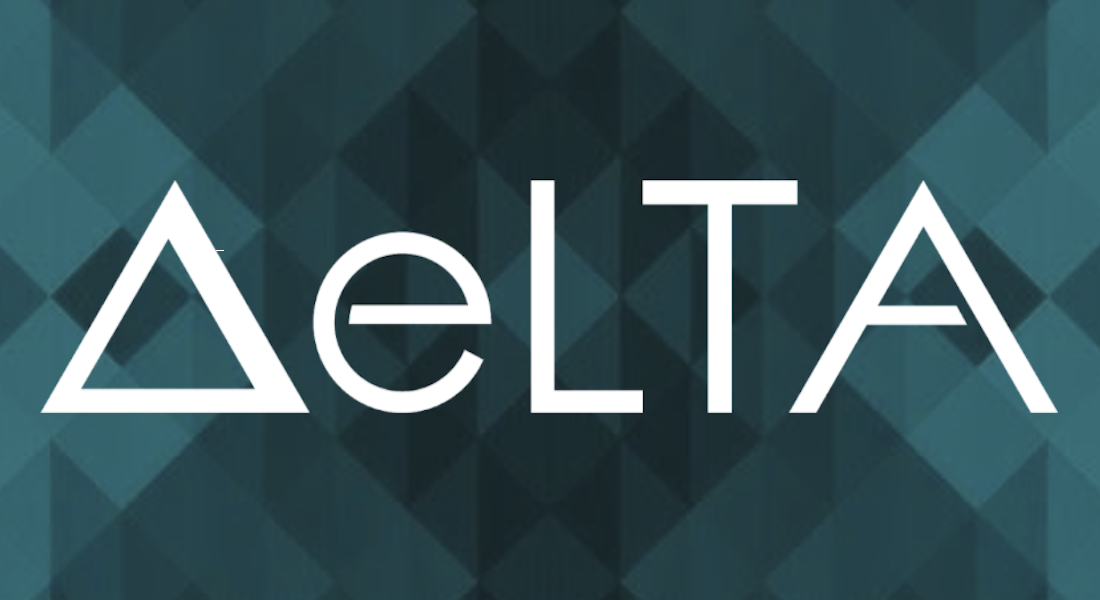
DeLTA is a research group affiliated with the Department of Computer Science at the University of Copenhagen studying diverse aspects of Machine Learning Theory and its applications, including, but not limited to Reinforcement Learning, Online Learning and Bandits, PAC-Bayesian analysis