Artificial intelligence may be the secret weapon in the climate fight
Massive climate change alters the world’s ecosystems every day, but we lack effective digital tools to monitor, simulate and predict events. Two SCIENCE professors – from Computer Science and Geosciences – have joined forces to develop a method designed to improve our understanding of the consequences of climate change on our ecosystems.
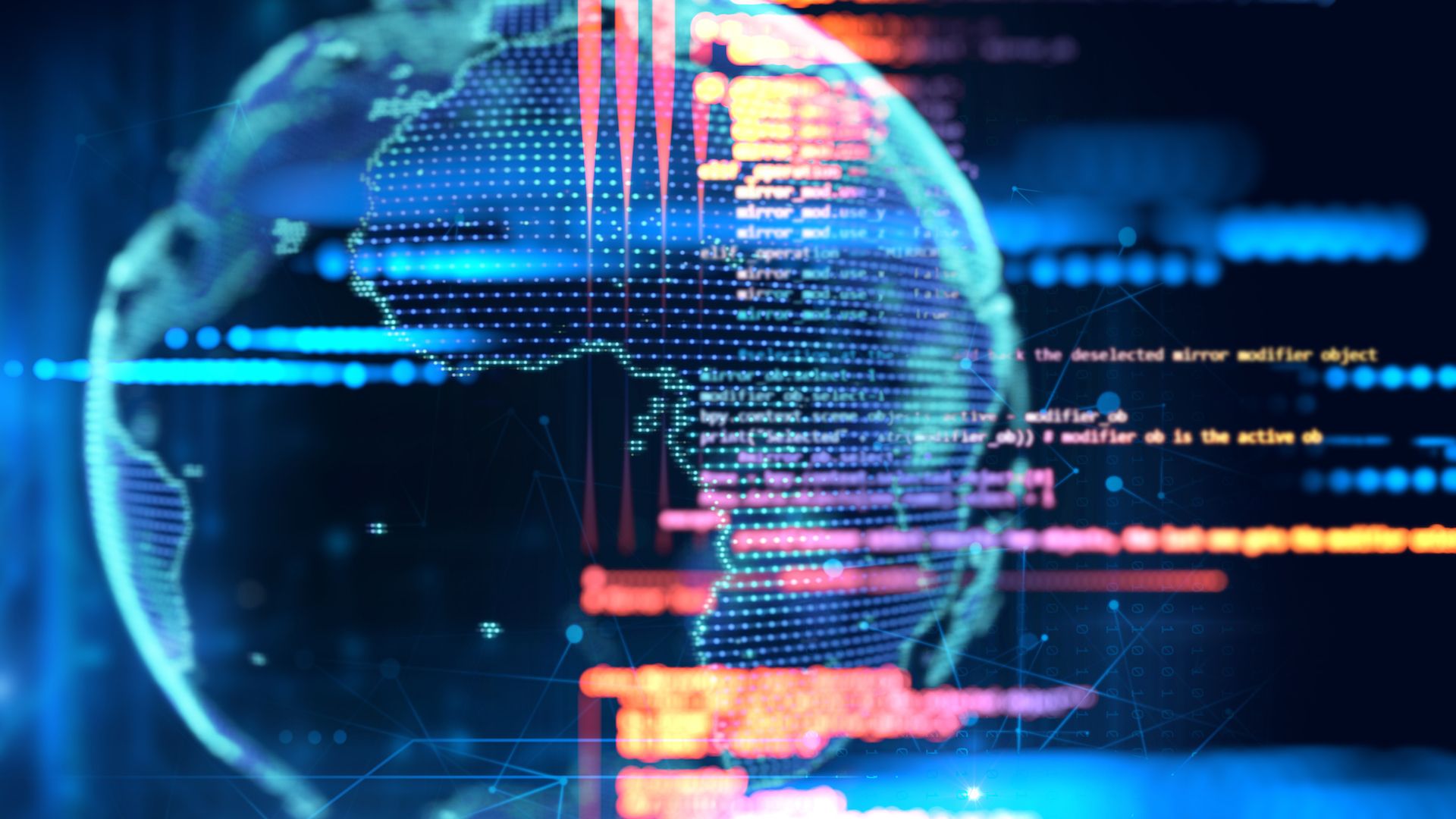
With almost DKK 15 million in funding from the VILLUM Foundation, Professor Christian Igel from the Department of Computer Science and Professor Rasmus Fensholt from the Department of Geosciences and Natural Resource Management are working together to develop new technologies to provide a better overview of the impact of climate change on the planet’s ecosystems.
“We don’t know enough about what climate change will really mean for the planet’s ecosystems and green resources in the future. Our new analytical models will help make knowledge acquisition far more effective. Better understanding is absolutely essential if we are to prevent and manage future changes as sustainably as possible, as formulated in the 17 UN Sustainable Development Goals,” says Rasmus Fensholt.
Four decades of satellite imagery
The new method combines artificial intelligence (AI) and satellite data. Based on technologies from both the Computer Science and Geosciences departments, the two researchers and their teams will work together to develop the best possible digital tool. Current geoscience analysis models are incapable of processing the large amounts of different types of satellite data to which we have access, making it difficult to use the information properly.
“Our goal is to take geoscience computational models to the next level by combining them with modern machine learning – in other words, algorithm-based artificial intelligence. Models based on machine learning are much more complex, and that makes them ideal for something as complex as the impact of climate change on our ecosystems,” explains Christian Igel.
Specifically, the method involves feeding the computer with satellite images taken over the last 40 years and containing information about the planet’s ecosystems and weather conditions. Based on many thousands of images, the researchers program the computer with algorithms that enables it to recognise patterns and correlations, and calculate future scenarios based on what it has learned. For example, the computer could be asked to count the number of trees in a specific area, identify the species, note whether a tree belongs to a plantation or natural forest, calculate the amount of carbon in an area, or study which of the planet’s forests can best withstand climate change – and which will not and will end up dying out.
The two researchers are particularly concerned with trees and forests, which cover 30% of Earth’s surface, house millions of animal and plant species, and are essential to the planet’s biodiversity. They also provide a livelihood for a large proportion of the human population, and their ability to absorb CO2 through photosynthesis plays a crucial role in combating climate change. Christian Igel and Rasmus Fensholt’s new research project will focus, among other things, on improved monitoring of the deterioration of the Earth’s vegetation resources due to deforestation and desertification caused by human over-exploitation or climate change.
Interdisciplinary synergy
By combining geoscience modelling with artificial intelligence’s ability to learn, recognise and anticipate patterns, the researchers aim to merge the best of two worlds. Geoscience researchers have traditionally used satellite measurements and scientific knowledge of ecosystems to simulate the current situation and predict the future. However, when it comes to climate change, this approach is inadequate, explains Christian Igel:
“At the moment, it’s impossible to make a detailed simulation of such a complex phenomenon as the effect of climate change on biodiversity and ecosystems. By using artificial intelligence, we can teach a computer to recognise patterns based on many years of data. Combining geoscience models and artificial intelligence gives us a much more precise view of the consequences of climate change, and a much better tool with which to face the future.”
The project will also make an important contribution to a number of general computer science questions that will be relevant to many other researchers. For example, it will explore how a computer can be taught to count the exact number of objects in an image. In an initial project, Christian Igel and Rasmus Fensholt, in collaboration with colleagues, have already developed a method that improves a computer’s ability to estimate the number of trees in Africa – including trees that are not in forests (e.g. savannah trees), but which are nevertheless essential to life for many people and animals on the planet.
The grant from the VILLUM FUND also allows researchers to purchase and test a new type of satellite data from micro-satellites (known as the cube rate) that enables the monitoring of global ecosystems based on highly detailed daily images. The project is named DeReEco (Deep Learning and Remote Sensing for Unlocking Global Ecosystem Resource Dynamics”) and is expected to be completed in 2026.
Related News
Contact
Christian Igel
Professor, Department of Computer Science
+45 21849673
igel@di.ku.dk
Caroline Wistoft
Communications Consultant, Department of Computer Science
+ 45 21309631
cawi@di.ku.dk
Machine learning and deep learning
Machine learning refers to the use of algorithms in artificial intelligence, i.e. machines being programmed to recognise patterns in data. Machine learning also utilises a number of methods called “deep learning”. Rasmus Fensholt and Christian Igel’s research project will combine these methods with data from satellite observation.
Deep learning is a more complex version of machine learning with bigger capacity to handle more complicated tasks. In a technique inspired by the human brain, it employs analysis based on several layers of computational processing. Deep learning has long been used, for example, in medical imaging such as MRI and X-ray scans, but it has never before been used to analyse satellite imagery and ecosystems.
Bio: Christian Igel
Christian Igel is a professor at the Department of Computer Science (DIKU) and head of the Science AI Centre – a multidisciplinary research centre in the Faculty of Science. The Centre’s main objective is to incorporate artificial intelligence techniques into various disciplines within the natural sciences, and thereby create new research opportunities. Christian’s main expertise is machine learning. He has been affiliated with DIKU’s Machine Learning Section for 10 years. Christian is originally from Germany. Before he arrived at DIKU, he worked as a junior professor at the Department of Neuroinformatics at Ruhr-University Bochum.
Bio: Rasmus Fensholt
Rasmus Fensholt is a professor in the Department of Geosciences and Nature Management (IGN) and part of the research group Environment and Society in Developing Countries. His focus is on remote sensing and time series of data from space satellites that monitor the Earth. Rasmus has an MSc in natural geography (1998) and a PhD (2004), both from the University of Copenhagen. He has been affiliated with the Department of Geosciences and Nature Management for 16 years. He started as a postdoc in 2004, during which time he, among other things, was affiliated with Goddard Space Flight Center at NASA. He then became assistant professor in 2007, associate professor in 2009 and professor in 2017.