DeLTA Seminar by Andres Masegosa
Speaker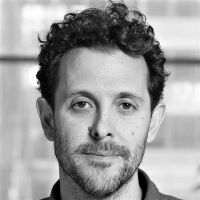
Andres Masegosa, Aalborg University.
Title
Diversity and Generalization in Neural Network Ensembles
Abstract
Ensembles are widely used in machine learning and, usually, provide state-of-the-art performance in many prediction tasks. From the very beginning, the diversity of an ensemble has been identified as a key factor for the superior performance of these models. But the exact role that diversity plays in ensemble models is poorly understood, specially in the context of neural networks. In this work, we combine and expand previously published results in a theoretically sound framework that describes the relationship between diversity and ensemble performance for a wide range of ensemble methods. More precisely, we provide sound answers to the following questions: how to measure diversity, how diversity relates to the generalization error of an ensemble, and how diversity is promoted by current neural network ensemble algorithms. This analysis covers three widely used loss functions, namely, the squared loss, the crossentropy loss, and the 0-1 loss; and two widely used model combination strategies, namely, model averaging and weighted majority vote. We empirically validate this theoretical analysis with neural network ensembles.
Andrés, L.A.O., Cabañas, R. and Masegosa, A. R., Diversity and Generalization in Neural Network Ensembles. In International Conference on Artificial Intelligence and Statistics (AISTATS 2022).
-------------------
You can subscribe to DeLTA Seminar mailing list by sending an empty email to delta-seminar-join@list.ku.dk.
Online calendar: https://calendar.google.com/calendar/embed?src=c_bm6u2c38ec3ti4lbfjd13c2aqg%40group.calendar.google.com&ctz=Europe%2FCopenhagen
DeLTA Lab page: https://sites.google.com/diku.edu/delta
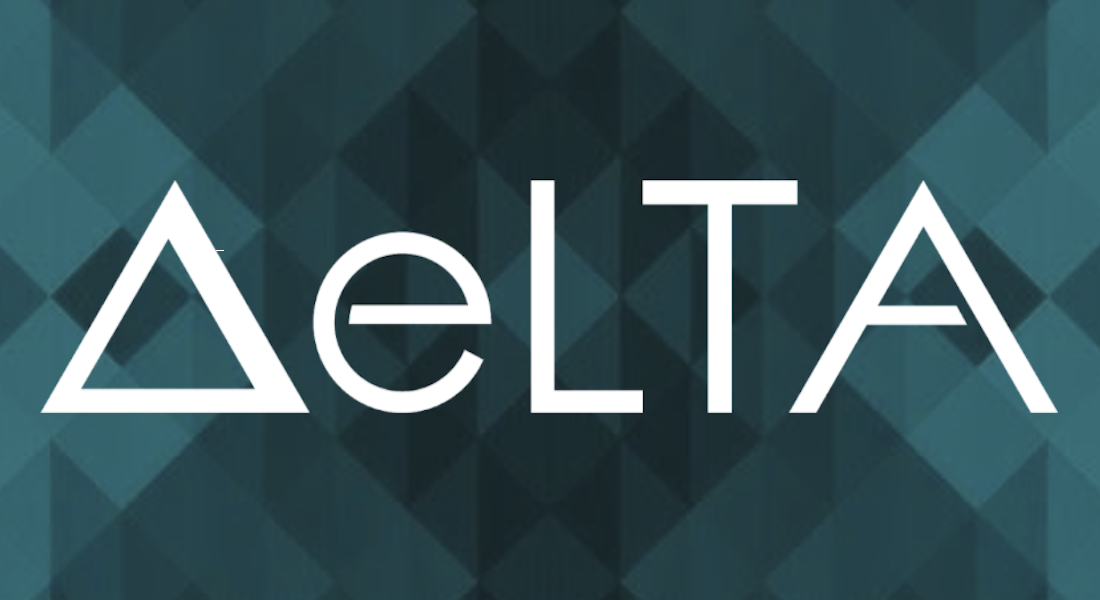
DeLTA is a research group affiliated with the Department of Computer Science at the University of Copenhagen studying diverse aspects of Machine Learning Theory and its applications, including, but not limited to Reinforcement Learning, Online Learning and Bandits, PAC-Bayesian analysis