DeLTA Seminar by Jalal Etesami
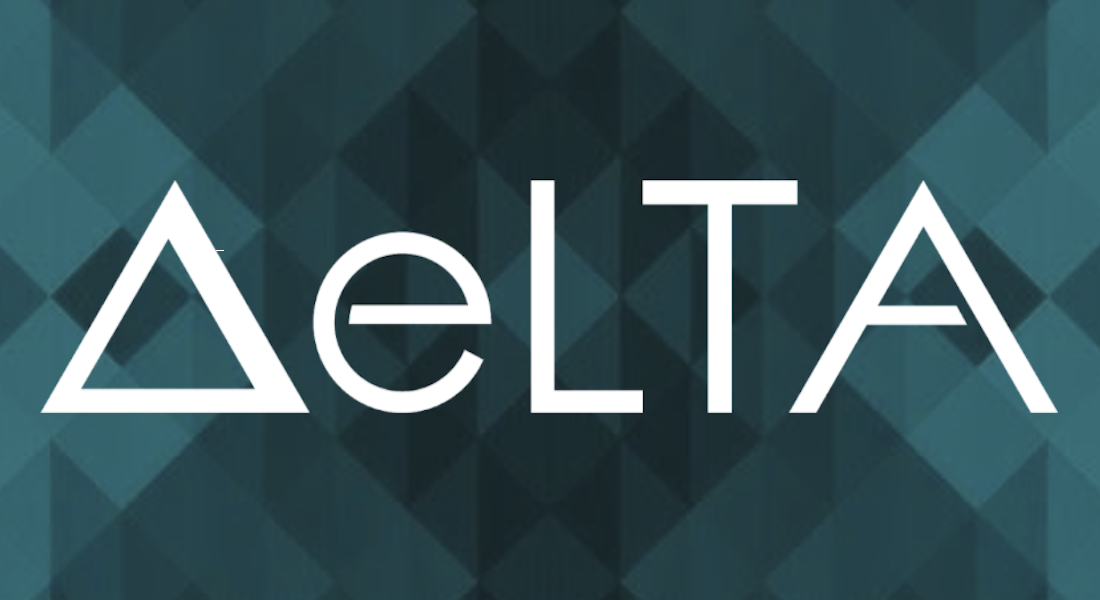
Speaker
Jalal Etesami
Title
Foundations of Causal Inference: Challenges and Opportunities
Abstract
Causal inference is a branch of machine learning and statistics that aims to develop theoretical models and practical algorithms to infer the statistical causal dynamics in complex systems. The incorporation of causality in learning is what predominantly sets human judgment apart from machines. Only when manmade systems acquire the ability to incorporate causal reasoning a true AI revolution will occur.
In this talk, I will briefly explain causal transfer learning and Granger’s notion of causality in time series and discuss how his formulation can go beyond linear dynamics. As a special case of causal inference in times series, I will focus on efficient algorithms for learning the causal relationships in a network of multivariate Hawkes processes. Such processes are a type of temporal marked point processes which are widely used in a variety of applications, e.g., analyzing epidemic pathways in global outbreaks of infectious diseases. Next, as a special case of causal transfer learning, I introduce causal imitation learning which is a powerful approach to train an AI agent in an environment by mimicking the behaviour of an expert in another environment.
-------------------
You can subscribe to DeLTA Seminar mailing list by sending an empty email to delta-seminar-join@list.ku.dk.
Information about DeLTA Seminars is available at the DeLTA Lab page: https://sites.google.com/diku.edu/delta
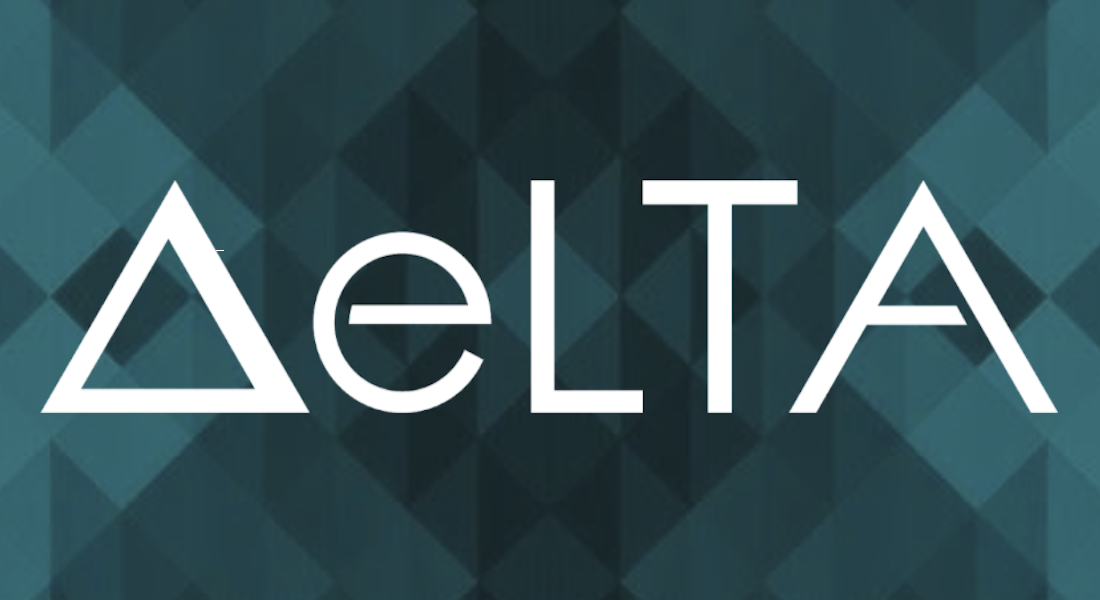
DeLTA is a research group affiliated with the Department of Computer Science at the University of Copenhagen studying diverse aspects of Machine Learning Theory and its applications, including, but not limited to Reinforcement Learning, Online Learning and Bandits, PAC-Bayesian analysis