DeLTA seminar by Saeed Masoudian
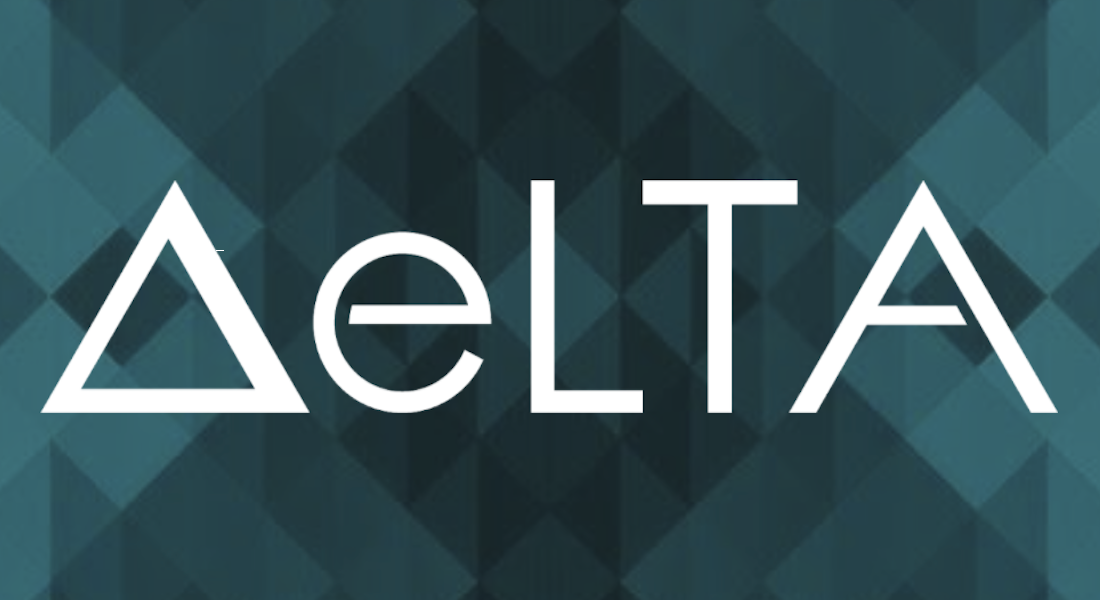
Speaker
Saeed Masoudian, Department of Computer Science, University of Copenhagen
Title
A Best-of-Both-Worlds Algorithm for Bandits with Delayed Feedback
Abstract
We present a modified tuning of the algorithm of Zimmert and Seldin (2020) for adversarial multiarmed bandits with delayed feedback, which in addition to the minimax optimal adversarial regret guarantee shown by Zimmert and Seldin simultaneously achieves a near-optimal regret guarantee in the stochastic setting with fixed delays.
Specifically, the adversarial regret guarantee is O(\sqrt{TK} + \sqrt{dT\log K}), where T is the time horizon, K is the number of arms, and d is the fixed delay, whereas the stochastic regret guarantee is O(\sum_{i \neq i^*} (\log(T)/\Delta_i + d/(\Delta_{i}\log K)) + d K^{1/3}\log K), where \Delta_i are the suboptimality gaps. We also present an extension of the algorithm to the case of arbitrary delays, which is based on an oracle knowledge of the maximal delay d_{max} and achieves O(\sqrt{TK} + \sqrt{D\log K} + d_{max}K^{1/3} \log K) regret in the adversarial regime, where D is the total delay, and O(\sum_{i \neq i^*} (\log(T)/\Delta_i + \sigma_{max}/(\Delta_{i}\log K)) + d_{max}K^{1/3}\log K) regret in the stochastic regime, where \sigma_{max} is the maximal number of outstanding observations. Finally, we present a lower bound that matches regret upper bound achieved by the skipping technique of Zimmert and Seldin (2020) in the adversarial setting.
Based on joint work with Julian Zimmert and Yevgeny Seldin.
Paper link: https://arxiv.org/abs/2206.14906
You can subscribe to the DeLTA Seminar mailing list by sending an empty email to delta-seminar-join@list.ku.dk.
Online calendar: https://calendar.google.com/calendar/embed?src=c_bm6u2c38ec3ti4lbfjd13c2aqg%40group.calendar.google.com&ctz=Europe%2FCopenhagen
DeLTA Lab page: https://sites.google.com/diku.edu/delta
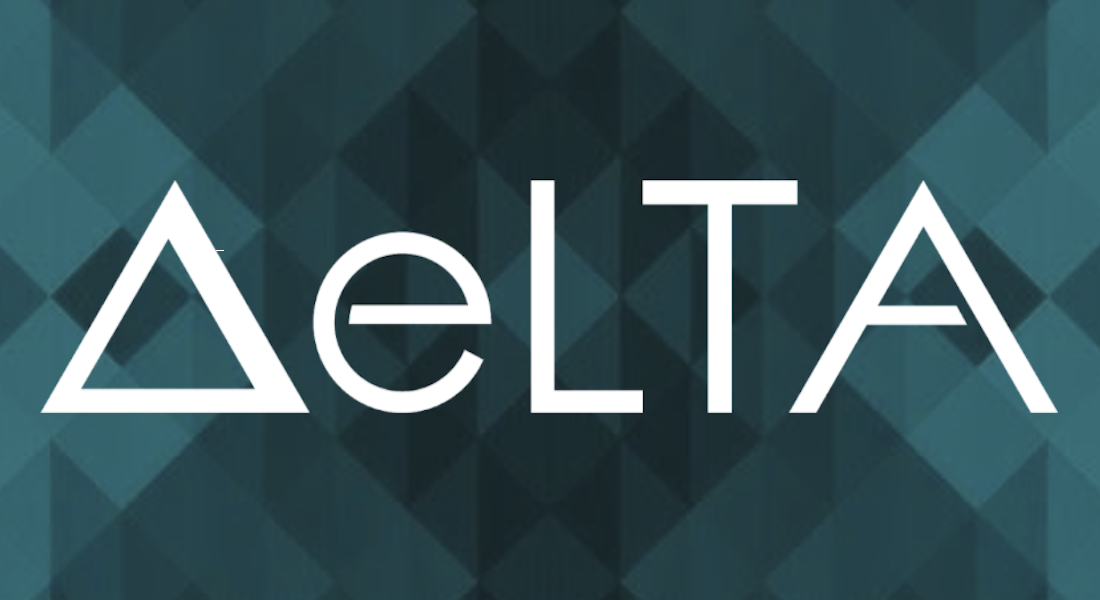
DeLTA is a research group affiliated with the Department of Computer Science at the University of Copenhagen studying diverse aspects of Machine Learning Theory and its applications, including, but not limited to Reinforcement Learning, Online Learning and Bandits, PAC-Bayesian analysis