DeLTA seminar by Badr-Eddine Chérief-Abdellatif : Bayes meets Bernstein in meta-learning
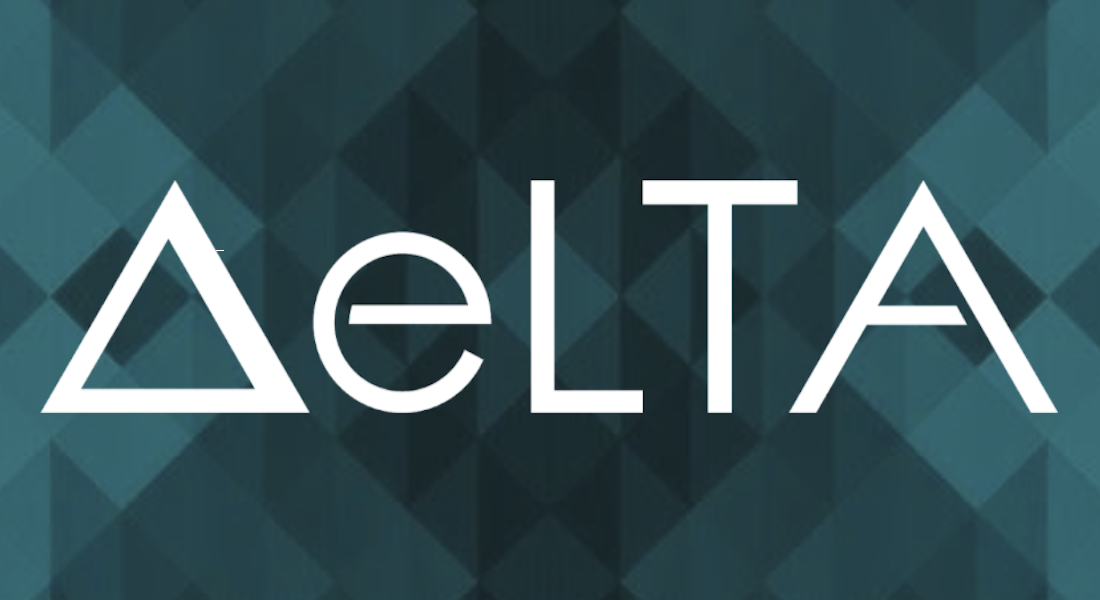
Speaker
Badr-Eddine Chérief-Abdellatif, CNRS researcher at Sorbonne Université & Université Paris Cité.
Title
Bayes meets Bernstein in meta-learning
Abstract
The rate of convergence of Bayesian learning algorithms is determined by two conditions: the probability mass given by the prior to neighborhoods of the optimal parameter (prior mass condition), and the behavior of the loss function around the optimal parameter (Bernstein condition). In meta-learning, we are faced with multiple learning tasks that are independent but expected to be related in some way. Our goal is to meta-learn a better prior that will be used to solve future tasks more efficiently. We propose to use PAC-Bayes in the meta-learning process and Bayesian inference at the within-task level, and prove that very fast convergence rates can be achieved by showing that: i) the prior mass condition can be improved in future task learning, and ii) the Bernstein condition is always satisfied at the meta-level.
_____________________________
You can subscribe to the DeLTA Seminar mailing list by sending an empty email to delta-seminar-join@list.ku.dk.
Online calendar
DeLTA Lab page
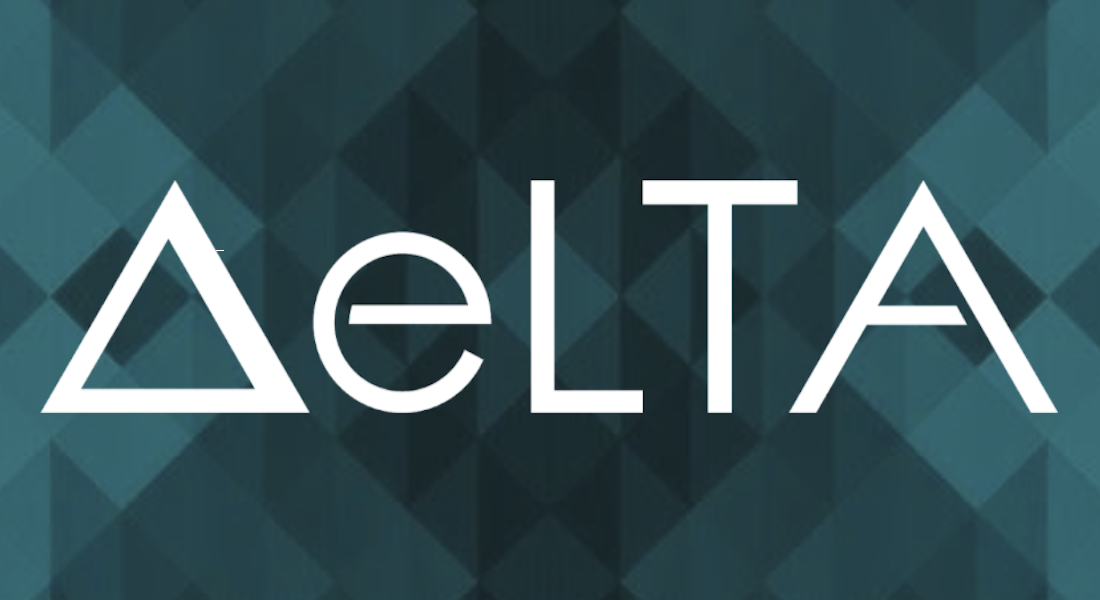
DeLTA is a research group affiliated with the Department of Computer Science at the University of Copenhagen studying diverse aspects of Machine Learning Theory and its applications, including, but not limited to Reinforcement Learning, Online Learning and Bandits, PAC-Bayesian analysis