DeLTA seminar by Julian Zimmert
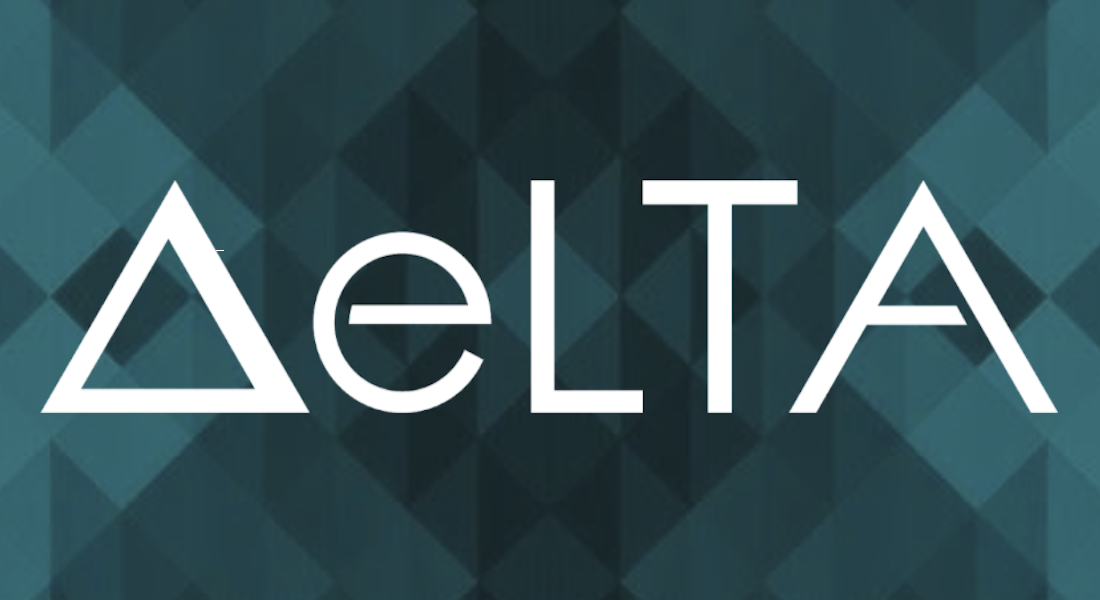
Speaker
Julian Zimmert, Google Research
Title
A Blackbox Approach to Best of Both Worlds in Bandits and Beyond
Abstract
Best-of-both-worlds algorithms for online learning which achieve near-optimal regret in both the adversarial and the stochastic regimes have received significant attention in the recent past. Existing techniques require careful adaptation to every new problem setup, including specialised potentials and careful tuning of algorithm parameters. In this work, we present a general reduction that allows us to obtain best-of-both-worlds guarantees for a wide family of follow-the-regularized-leader (FTRL) algorithms. We showcase the capability of this reduction by transforming existing algorithms, only known to achieve worst-case guarantees, into new algorithms with best-of-both-worlds guarantees in contextual bandits, graph bandits and tabular Markov decision processes.
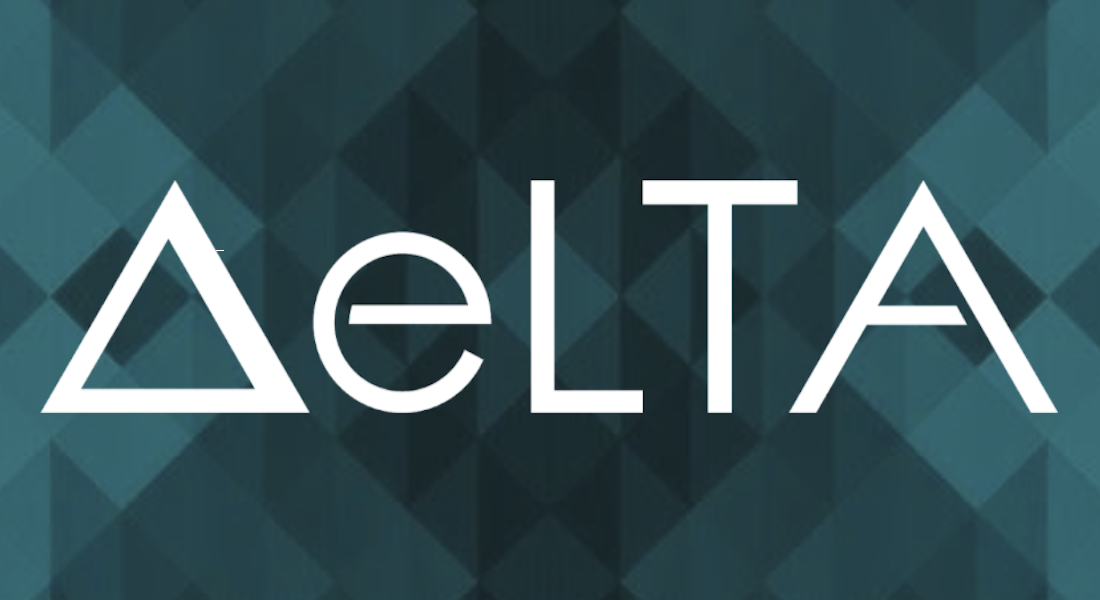
DeLTA is a research group affiliated with the Department of Computer Science at the University of Copenhagen studying diverse aspects of Machine Learning Theory and its applications, including, but not limited to Reinforcement Learning, Online Learning and Bandits, PAC-Bayesian analysis