DeLTA seminar by Kaixin Wang: Laplacian Representation in Reinforcement Learning: Approximation, Reachability, and Beyond
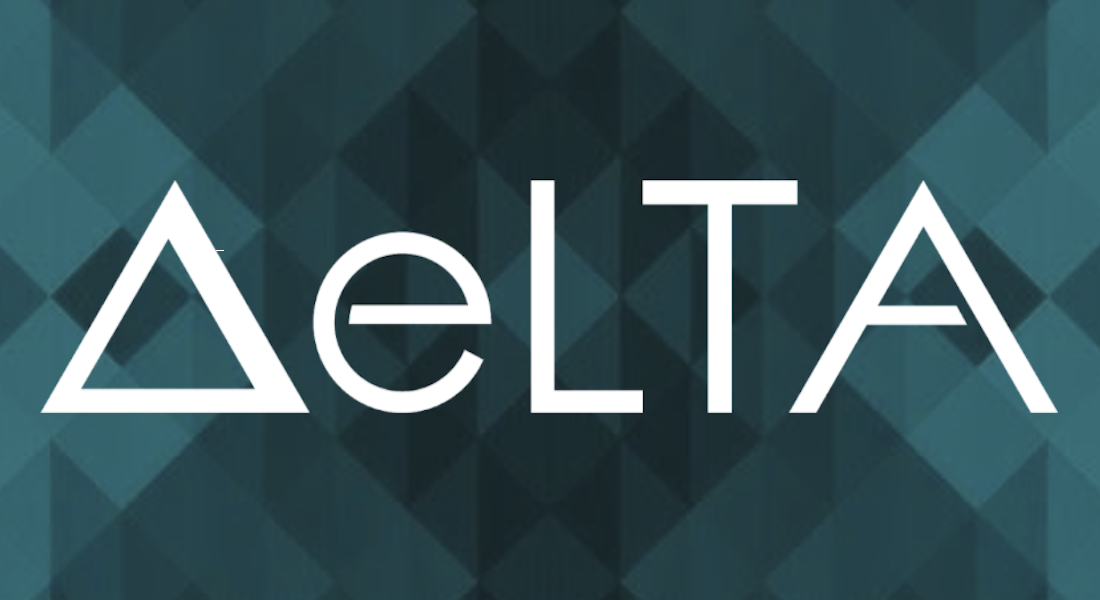
Speaker
Kaixin Wang, postdoctoral researcher at the Technion
Title
Laplacian Representation in Reinforcement Learning: Approximation, Reachability, and Beyond
Abstract
Laplacian representation is a task-agnostic state representation in reinforcement learning (RL), which aims to capture the geometric properties of the environment and is independent of the task reward. In this talk, I will first introduce a generalized graph drawing method for better approximating the Laplacian representation with neural networks. Building on this method, I will present how we can correctly measure the inter-state reachability with the learned Laplacian representation. Finally, I will discuss some interesting directions in this field.
Bio
Kaixin Wang is a postdoctoral researcher in the Faculty of Electrical and Computer Engineering at the Technion, working with Shie Mannor. Prior to that, he received his Ph.D. degree from the Institute of Data Science at the National University of Singapore, under the supervision of Jiashi Feng, Bryan Hooi and Xinchao Wang. His research interests include representation learning, generalization, and robustness in reinforcement learning.
_____________________________
You can subscribe to the DeLTA Seminar mailing list by sending an empty email to delta-seminar-join@list.ku.dk.
Online calendar
DeLTA Lab page
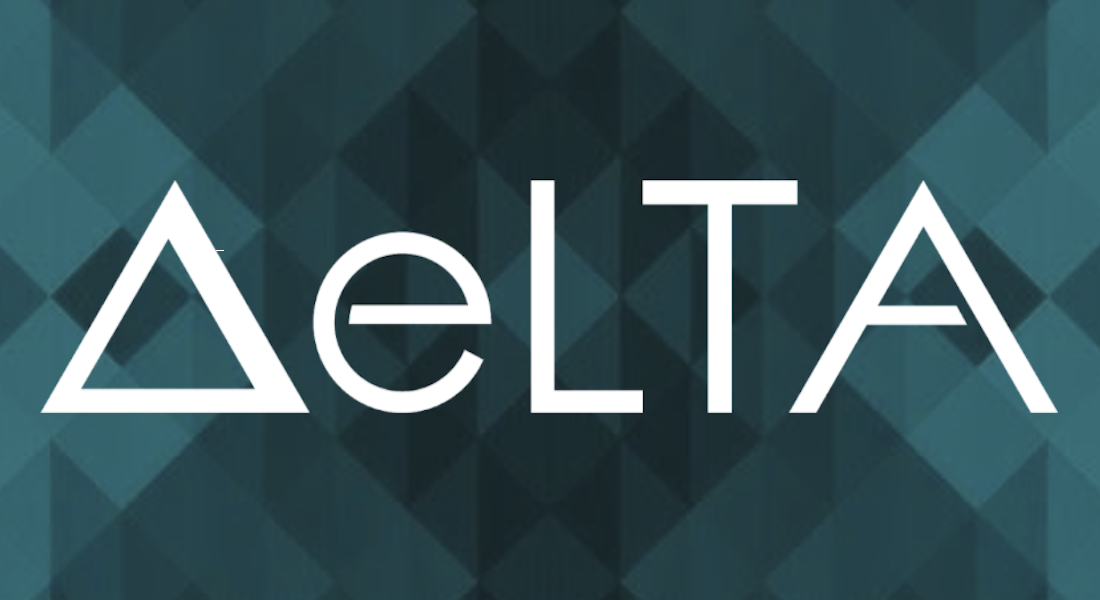
DeLTA is a research group affiliated with the Department of Computer Science at the University of Copenhagen studying diverse aspects of Machine Learning Theory and its applications, including, but not limited to Reinforcement Learning, Online Learning and Bandits, PAC-Bayesian analysis