DeLTA seminar by Yijun Bian: Study of Fair ML Models from a Theoretical Perspective
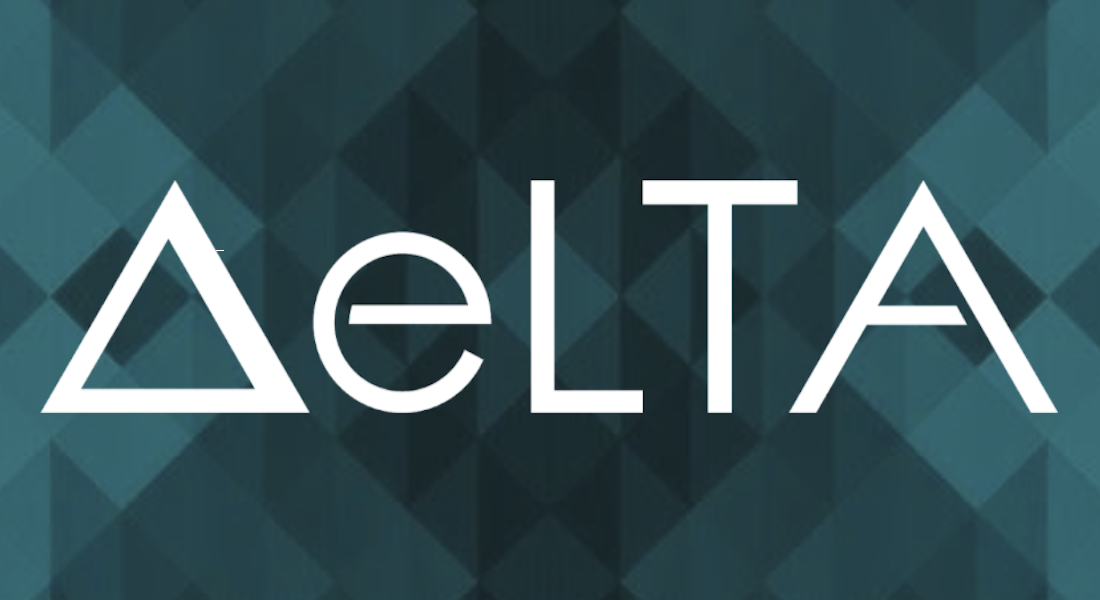
Follow this link to participate on Zoom
Speaker
Yijun Bian, DIKU (ML section)
Title
Study of Fair ML Models from a Theoretical Perspective
Abstract
Given various machine learning (ML) applications in the real world, concerns about discrimination and unfairness hidden in ML models are growing, particularly in high-stakes domains. Yet the existing fairness measures for assessing the discrimination level of ML models are usually incompatible with each other, meaning that unfairness may still exist even when some fairness measures are satisfied. Moreover, few of them can deal with scenarios where more than one sensitive attribute with multiple potential values exists. In this talk, I will discuss two types of fairness measures for the multiple sensitive attributes' scenario: one, named discriminative risk, captures aspects from both individual and group fairness; the other evaluates the added discrimination introduced in the learning process (on top of potential discrimination present in the training data), by viewing instances with sensitive attributes as data points on certain manifolds. I will further discuss how we can use the two fairness measures to provide fairness guarantees, to design fairer ensemble classifiers, and to efficiently evaluate the added discrimination introduced in the learning process. The proposed metrics can be used to mitigate discrimination in presence of multiple sensitive attributes, providing broad applicability.
_____________________________
You can subscribe to the DeLTA Seminar mailing list by sending an empty email to delta-seminar-join@list.ku.dk<mailto:delta-seminar-join@list.ku.dk>.
Online calendar: https://calendar.google.com/calendar/embed?src=c_bm6u2c38ec3ti4lbfjd13c2aqg%40group.calendar.google.com&ctz=Europe%2FCopenhagen
DeLTA Lab page: https://sites.google.com/diku.edu/delta