Final Appraisal Seminar for Tenure Track Assistant Professor Oswin Krause
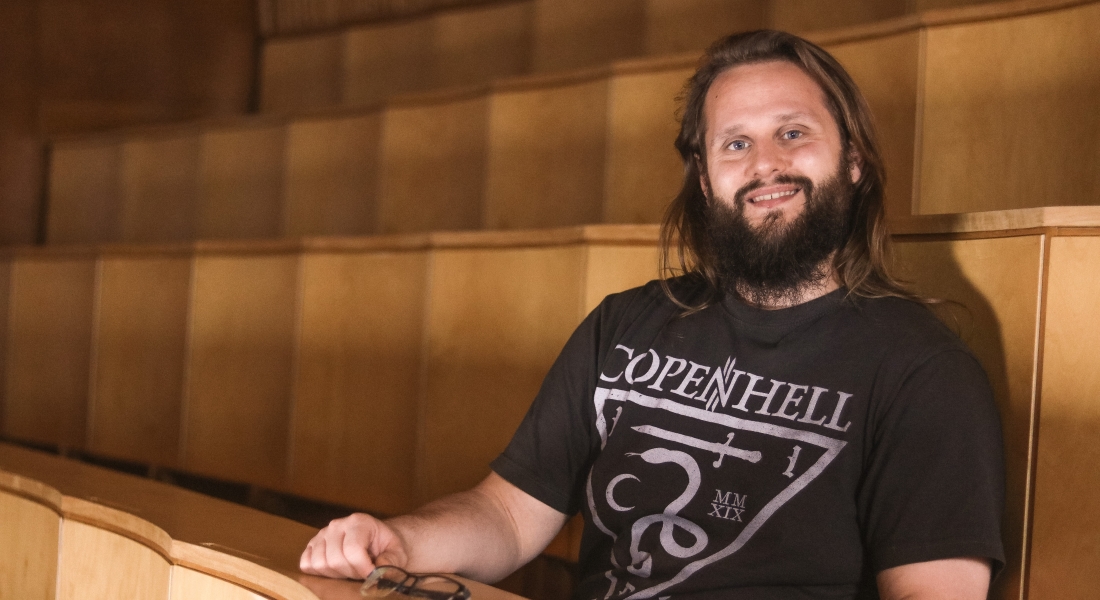
Program
- 13.25-13.30: Short welcome and introduction by the associate dean for research
- 13.30-14.15: 45 min presentation by the candidate consisting of the following:
- Presentation of the research conducted during the tenure track period and its relation to the state of the art (about 30 minutes)
- Presentation of future research visions (about 7-8 minutes)
- Reflections about teaching experience and presentation of visions for future teaching development (about 7-8 minutes)
- 14.15 – 14.20: Short break
- 14.20 – 14.40: Research questions by external opponent, Associate Professor Jes Frellsen
- 14.40 – 15:00: Research questions by external opponent, Professor Bernhard Sendhoff
- 15:00 – 15.15: Questions to teaching experience and visions by deputy head for education, Ken Friis Larsen
- 15.15 – 15.30: Questions regarding future ideas and visions, collaboration, international networking etc. by head of department and associate dean for research
- 15.30 – 15.45: Evaluation by Tenure Track appraisal-board (closed session - NB – in zoom breakout room - candidate and the audiences stays in main room)
- 15.45 – 16:00: Concluding evaluation and conversation between candidate and assessment board in front of the whole audience.
- 16:00 – 17:00: Reception with cake and bubbles
Title
Applied Machine Learning in the sciences
Abstract
Machine learning has left its traditional domains of audial and visual pattern recognition problems and its methods are now applied to various problems in the natural and medical sciences. On this boundary, the methods developed on clean benchmarks collide with the messy details of the real world and much of my research was focused on the problems arising here. In this talk I will report on two of my coiontributions:
In astrophysics, ground truth data is often sparse, expensive, or has large uncertainties, while observational data is often unstructured and incomplete due to limited observation time. Thus, it is important that our developed methods can not only handle this structural problems, but also reflects the uncertainty of the predictions: while each single prediction might be uncertain, the sum over uncertain predictions of thousands of observations might still allow to test physical theories. And thus, well-calibrated models are needed.
Another domain I heavily contributed to was establishing control of spin-qubit devices, where the machine learning tasks get complicated by the lack of clean sensor signals and the complete absence of data: a machine learning method must not only learn and predict from data, but also decide which data to collect.