PhD defence by Pepa Kostadinova Atanasova
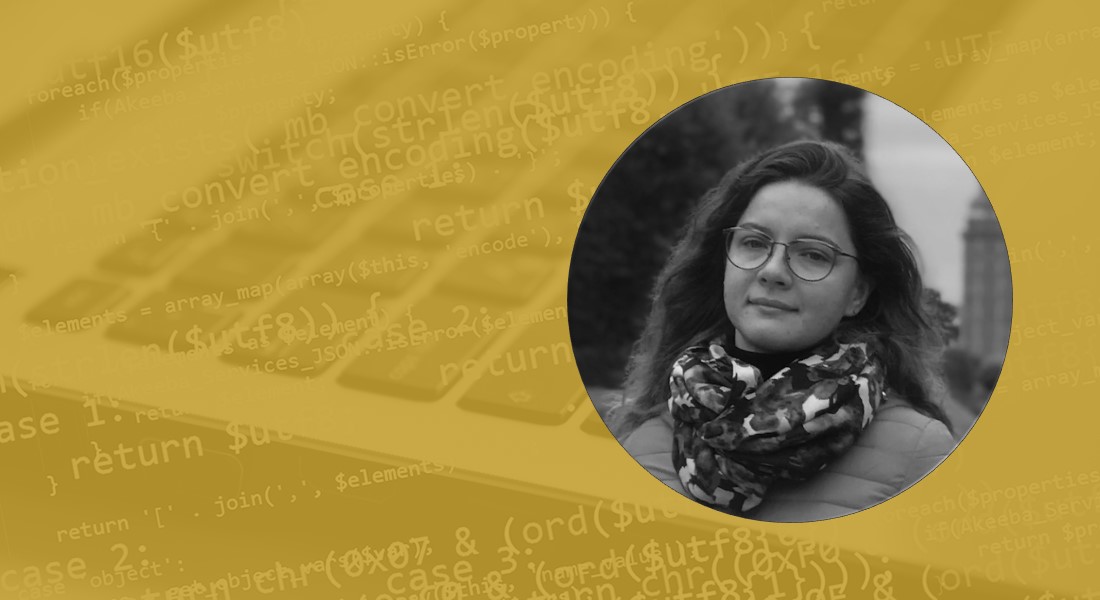
Title
Accountable and Explainable Methods for Complex Reasoning over Text
Abstract
A major concern of Machine Learning (ML) models is their opacity. They are deployed in an increasing number of applications where they often operate as black boxes that do not provide explanations for their predictions. Among others, the potential harms associated with the lack of understanding of the models' rationales include privacy violations, adversarial manipulations, and unfair discrimination. As a result, the accountability and transparency of ML models have been posed as critical desiderata by works in policy and law, philosophy, and computer science.
In computer science, the decision-making process of ML models has been studied by developing accountability and transparency methods. Accountability methods, such as adversarial attacks and diagnostic datasets, expose vulnerabilities of ML models that could lead to malicious manipulations or systematic faults in their predictions. Transparency methods explain the rationales behind models' predictions gaining the trust of relevant stakeholders and potentially uncovering mistakes and unfairness in models' decisions. To this end, transparency methods have to meet accountability requirements as well, e.g., being robust and faithful to the underlying rationales of a model.
This thesis presents my research that expands our collective knowledge in the areas of accountability and transparency of ML models developed for complex reasoning tasks over text. First, this thesis contributes with two methods for accountable ML models. They generate adversarial inputs and a diagnostic dataset demonstrating significant model vulnerabilities and suggesting ways to correct those. In the area of transparency of ML models, this thesis advances the state-of-the-art with methods generating textual explanations that are further improved to be fluent, easy to read, and to contain logically connected multi-chain arguments. Finally, this thesis makes contributions in the area of diagnostics for explainability approaches with a set of properties for evaluating existing explainability techniques and methods for enhancing those further in produced explanations. All of the contributions are empirically tested on complex reasoning tasks over text, including fact checking, question answering, and natural language inference.
Supervisors
Professors Isabelle Augenstein (Principal supervisor), Jakob Grue Simonsen, Christina Lioma
Assessment Committee
Chairperson: Professor Igel Christian, Department of Computer Science, University of Copenhagen
Reader Ivan Titov, University of Edinburgh
Professor Kalina Bontcheva, The University of Sheffield
Leader of defence: Tenure-track Assistant Professor Maria Maistro
For a digital copy of the thesis, please visit https://di.ku.dk/english/research/phd/
Join as Zoom Meeting:
https://ucph-ku.zoom.us/j/63869091763?pwd=RDl2dEVoVnR4Wk0xYzV5QWtNeGRLZz09
With passcode:
982580