PhD defence by Abraham George Smith
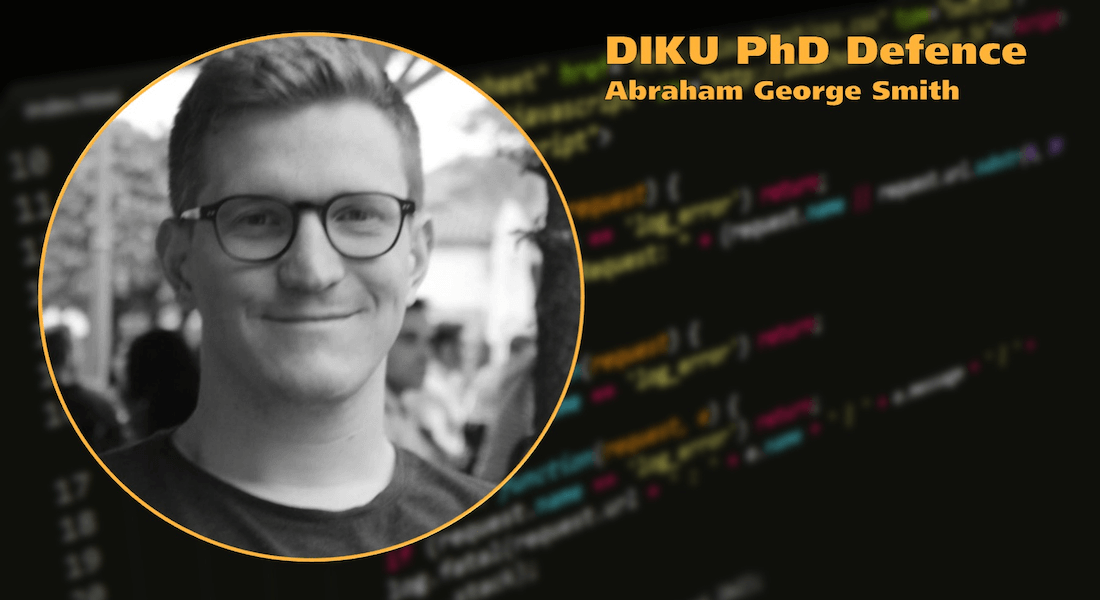
Title
Deep-Learning Image Segmentation with Corrective Annotation
Abstract
Segmentation involves automatically drawing objects in images and is relevant to a broad range of fields such as agriculture and medicine. Although AI methods allow segmentation to be performed automatically, data labelling and model training are still cumbersome tasks involving technical expertise.
This thesis presents an investigation into the effectiveness of IML (interactive machine learning) for the rapid training of AI segmentation models by non-expert users for use in both plant science and radiotherapy.
Using multiple segmentation tasks it was found that IML via corrective-annotation provides a way to rapidly train fully-automatic deep-learning models for a variety of datasets. The IML process can also be directly used for completing tasks, with the model learning from human feedback.
The IML methods in the thesis are evaluated using real-world segmentation projects with real human annotators, enabling translation to several subsequent image-analysis-based research projects in various domains.
Supervisors
Principal Supervisor Jens Petersen
Assessment Committee
Professor Mads Nielsen, Department of Computer Science
Senior Lecturer Marianne Aznar, Radiotherapy Related Research, University of Manchester
Associate Professor Dimitrios Papadopoulos, DTU Compute, Technical University of Denmark
For an electronic copy of the thesis, please visit the PhD Programme page.