PhD defence by Christian Thygesen
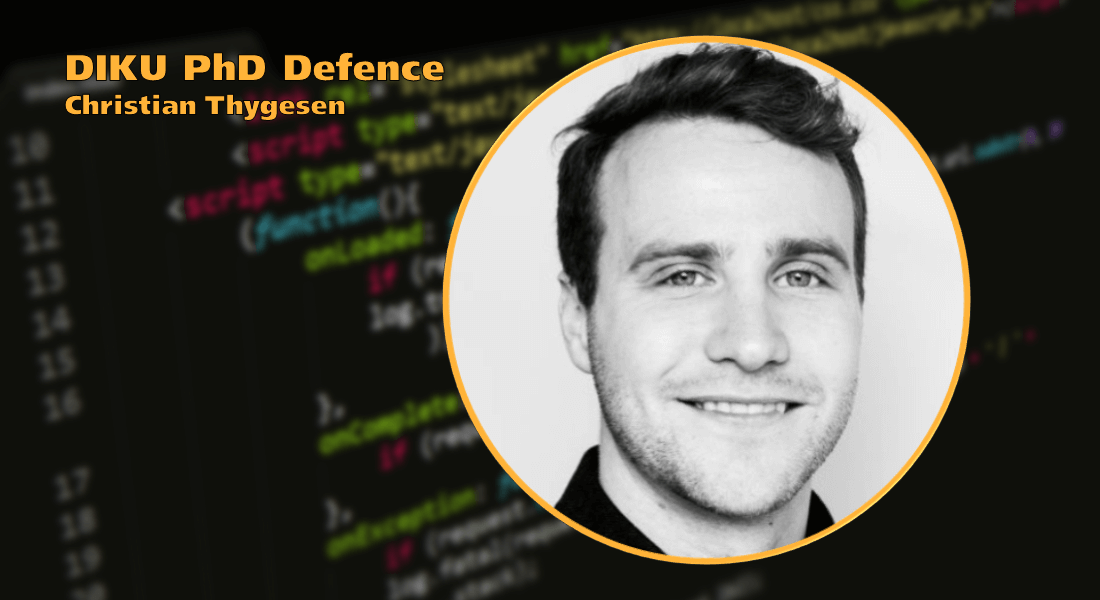
Title
Accelerating vaccine development through a deep probabilistic programming approach to protein structure prediction
Abstract
This dissertation aims to study the use of deep probabilistic programming for protein structure prediction and subsequently vaccine design.
The first paper presents a novel deep and generative Markov model for prediction of local protein structure. The model allows sampling of the backbone dihedral angles of protein structure fragments given only their amino acid sequence. It performs on par with the state-of-the-art at a greatly improved runtime using a fraction of the same prior information.
The second paper is an application of said model in a vaccine design setting. We deploy the model as a similarity function, allowing us to arbitrarily change stretches of the amino acid sequence of a protein, while maintaining the structural fold. We use it to enrich an existing vaccine product with immune epitopes from foreign sources, thus potentially increasing the efficacy and broadness of the vaccine.
The third and final paper presents a novel approach to train models of protein structure by introducing likelihoods over both the backbone dihedral angles as well as the 3D-coordinates. We show that training a model with this combined likelihood we achieve better performance on small protein fragments, while simultaneously allowing us to model much longer amino acid sequences.
Supervisors
Principal Supervisor Thomas Wim Hamelryck
Co-Supervisor Anders Bundgaard Sørensen
Assessment Committee
Associate Professor Wouter Boomsma, Department of Computer Science, UCPH
Professor Morten Nielsen, Technical University of Denmark
Assistant Professor Simon Olsson, Chalmers University of Technology, Gothenburg, Sweden
Leader of defence: Associate Prof. Wouter Boomsma, Department of Computer Science, UCPH
For an electronic copy of the thesis, please visit the PhD Programme page.