PhD defence by Hubert Dariusz Zając
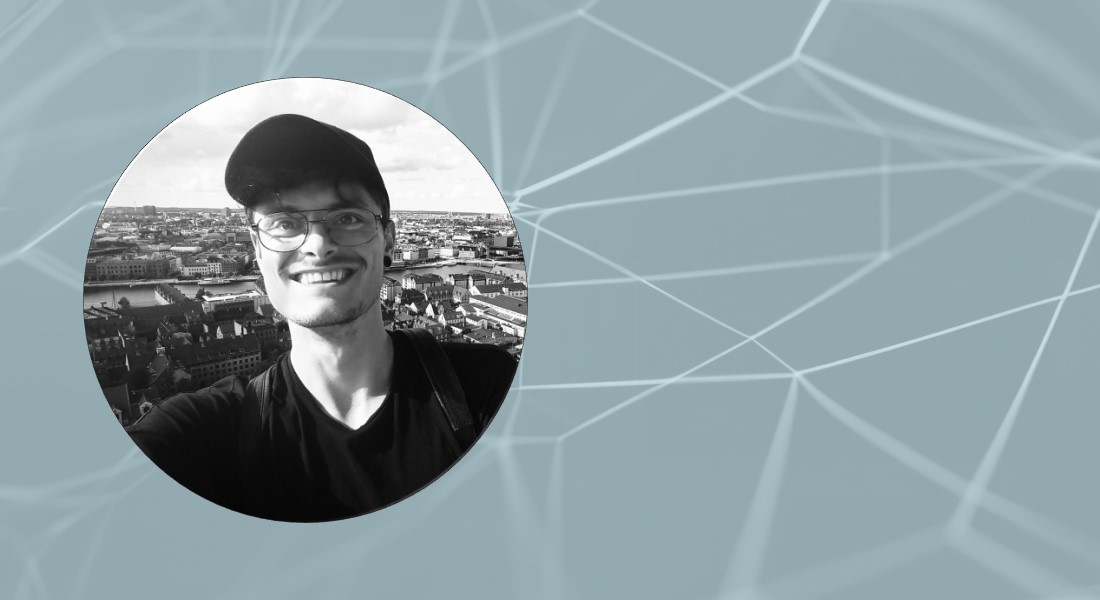
Title
Abstract
Artificial Intelligence (AI) in healthcare, especially based on Machine Learning (ML) techniques, holds significant promise for the field. These techniques have been extensively applied to address various clinical challenges, including pathology detection in X-rays, CT and MRI scans, mammography, skin cancer detection, diabetic retinopathy identification, and predicting readmissions and post-surgery complications. However, despite these advancements, AI-based systems remain notably absent in current clinical practice, limiting their clinical impact. One key factor contributing to this gap is the prevalent technology-centric approach to AI innovation, which often results in the limited clinical usefulness of AI-based support systems.
In this thesis, part of the AI4XRAY project (2020-2025), I explore the design and development of AI-based systems useful for medical professionals. Employing a combination of literature review, ethnographic research, and design work, I investigate the clinical usefulness of AI-based tools, focusing on chest X-ray support for radiologists in Denmark and Kenya.
Key findings from this research include:
- Enriched conceptualisation of clinical usefulness, highlighting the importance of end-user expectations, pre-labelling of medical datasets, and broadening the design space for AI systems to enhance organisational acceptance and clinical efficacy.
- Identification of sociotechnical challenges hindering the implementation of clinical AI, emphasising the need for collaboration across disciplines to address technical and social complexities.
- Emphasis on the pre-labelling phase in dataset creation, acknowledging external and internal factors that shape data collection, usage, and ground truth schemas, ultimately influencing the design space of future AI systems.
- Proposal of five practical visions for AI support in chest X-ray practice, addressing real-world challenges and offering insights beyond traditional technology-centred approaches.
- Advocacy for configuring AI systems to align technical dimensions with clinical needs, considering factors such as medical knowledge, user expertise, and patient context. This alignment ensures value delivery in clinical practice and emphasises the importance of ongoing consideration of dependencies in system configuration.
By addressing these key points, this thesis contributes to understanding the clinical usefulness of AI in healthcare and advocates for interdisciplinary collaboration to realise the full potential of AI-based systems in clinical practice.
Supervisors
Principal Supervisor: Associate Professor Tariq Osman Andersen
Co-Supervisor: Professor Emeritus Finn Kensing
Assessment Committee
Associate Professor Naja Holten Møller, DIKU
Professor Margot Brereton, Queensland University of Technology
Associate Professor Niels van Berkel, Aalborg University, Human-Centered Computing Group
Leader of defense: Tenure Track Assistant Professor Sarah Frances Homewood, DIKU
For an electronic copy of the thesis, please visit the PhD Programme page.