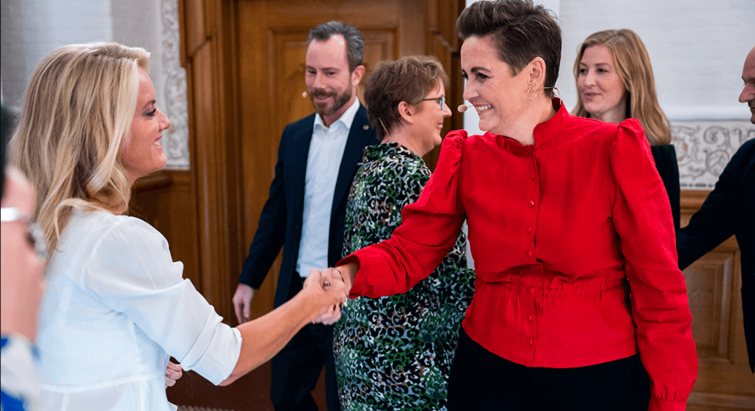
PhD defence by Karolina Stańczak
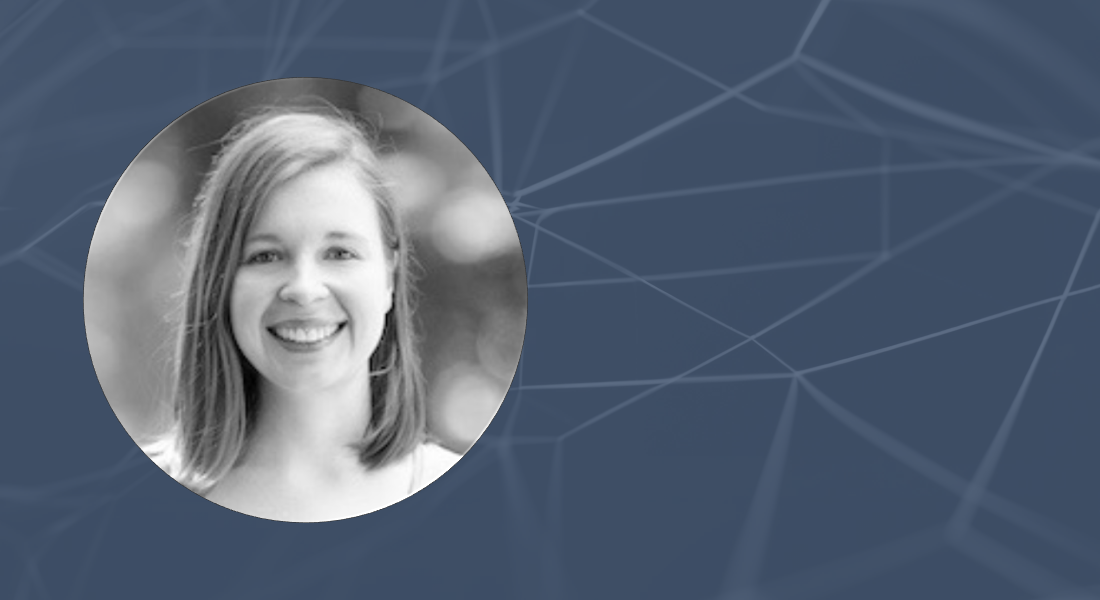
Title
A Multilingual Perspective on Probing Gender Bias
Abstract
Gender bias represents a form of systematic negative treatment that targets individuals based on their gender. This discrimination can range from subtle sexist remarks and gendered stereotypes to outright hate speech. Prior research has revealed that ignoring online abuse not only affects the individuals targeted but also has broader societal implications. These consequences extend to the discouragement of women’s engagement and visibility within public spheres, thereby reinforcing gender inequality. This thesis investigates the nuances of how gender bias is expressed through language and within language technologies. Significantly, this thesis expands research on gender bias to multilingual contexts, emphasising the importance of a multilingual and multicultural perspective in understanding societal biases. In this thesis, I adopt an interdisciplinary approach, bridging natural language processing with other disciplines such as political science and history, to probe gender bias in natural language and language models. In the area of natural language processing, this thesis has led to the curation of datasets derived from different domains, including social media data and historical newspapers, to analyse gender bias. The methodological contributions presented in my thesis include introducing measures of intersectional biases in natural language, and a causal study of the influence of a noun’s grammatical gender on people’s perception of it. In the area of probing methods for language models, this thesis introduces novel methods for probing for linguistic information and societal biases encoded in their representations. The contributions include two distinct methodologies for dataset creation. The first methodology employs a simple template structure that allows for generating words directly next to entity names to measure language models’ associations with these entities. The second involves collecting stereotypes and a set of identities belonging to different societal categories to comprise a probing dataset to analyse language models’ associations with societal groups, and identities within these groups. The methodological contributions range from a latent-variable model designed for probing linguistic information to a novel measure for identifying broader societal biases beyond gender. Taken together, this thesis has contributed to advancing our understanding of methodologies for analysing as well as the prevalence of gender bias in both natural language and language models.
Supervisors
- Advisor: Isabelle Augenstein, Department of Computer Science, University of Copenhagen
- Co-advisor: Ryan Cotterell, ETH Zurich
Assessment Committee
- Full Professor: Serge Belongie, Department of Computer Science, University of Copenhagen (Leader of defence)
- Chair Professor: Pascale Fung, The Hong Kong University of Science and Technology
- Principal Research Associate: Ivan Vulic, University of Cambridge & PolyAI
For an electronic copy of the thesis, please visit the PhD Programme page.
Related article
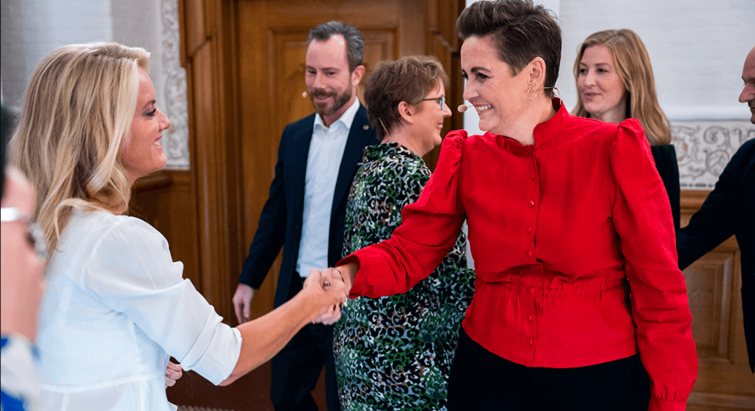