PhD defence by Paul Schreiner
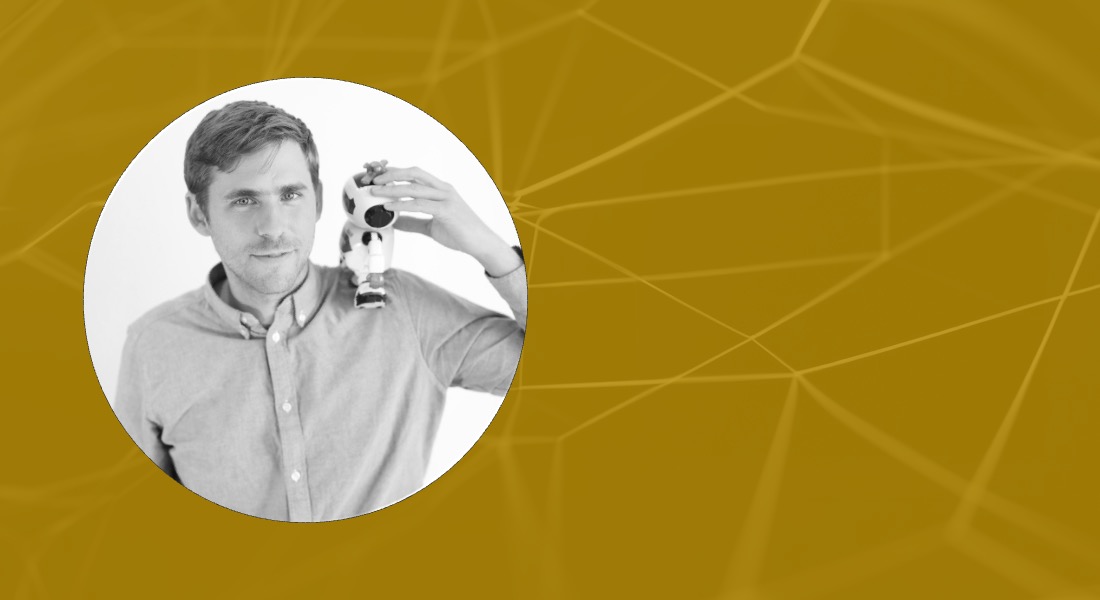
Title
ADAM: Auto-Animation of Digital Humans Using AI Methods
Abstract
IMU based motion capture data notoriously lacks global positional information, due to inherent limitations in the sensing hardware. On top of that, it often suffers from physically implausible data artefacts, such as interpenetration of body parts, penetration of the floor and foot skating. The research in this thesis presents methods for reconstructing global position trajectories from local pose information and improving IMU motion data quality in an automated fashion. The first phase of this work introduces a novel method for reconstructing global position trajectories from short sequences of pose information using a U-Net convolutional neural network. Building upon this foundation, a method is then introduced that includes acceleration signals to the training data, resulting in accurate trajectories even when pose data quality deteriorates. In contrast to the previous work, this method includes vertical displacement estimation. Tested on a dataset comprising actors not included in the training set, this enhanced method showed good accuracy in reconstructing ground truth trajectories. Acceleration signals were shown to play a critical role in maintaining trajectory reconstruction quality when pose data quality declined.
The last part of this thesis tackled inherent limitations in IMU-based motion capture, such as self-penetrating body parts, foot skating, and floating. These issues significantly hamper the realism achievable with cost-effective IMU systems. To overcome this, reinforcement learning was utilised to train an AI agent that could mimic error-prone sample motions within a simulated environment. This approach could prevent these common distortions while preserving the unique characteristics of the sample motions. The agent was trained on a blend of faulty IMU data and high-quality optical motion capture data. The efficacy of this approach was validated employing a set of quantitative metrics. These tests, conducted on a benchmark dataset of IMU-based motion data from actors outside the training set, demonstrated the method’s capability to enhance the realism and usability of IMU-based motion capture systems, narrowing the gap with marker-based alternatives.
Supervisors
Principal Supervisor Kenny Erleben
Co-Supervisor Matias Søndergaard
Assessment Committee
Associate Professor Joanna Bergström, Department of Computer Science, UCPH
Professor Miguel A. Otaduy, Universidad Rey Juan Carlos
Associate Professor Sheldon Andrews, École de technologie supérieure
Leader of defence: Hang Yin, Department of Computer Science, UCPH
For an electronic copy of the thesis, please visit the PhD Programme page.