PhD defence by Andreas David Lauritzen
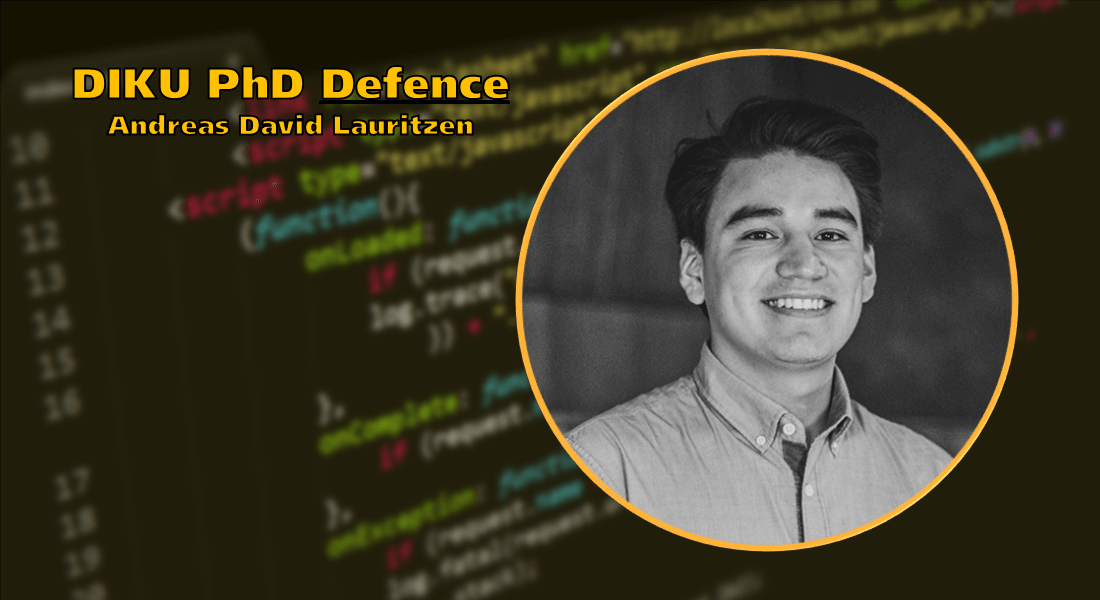
Title
Development and Clinical Evaluation of Deep Learning Models for Personalized Breast Cancer Screening
Abstract
Breast cancer is the single most diagnosed type of cancer among women worldwide. Personalized mammography screening protocols, tailored to the individual woman's risk of a future breast cancer, might further improve screening quality, and facilitate better exploitation of radiologists' resources.
This thesis aimed at developing and evaluating mammography-based breast cancer risk models that might aid clinicians in implementing personalized screening strategies.
An artificial intelligence (AI)-based screening protocol was simulated and showed a 63% reading workload reduction for breast radiologists while preserving high screening quality. This simulation study contributed to a clinical implementation of AI in the Capital Region of Denmark breast cancer screening program.
A mammographic texture risk model was developed trained to extract textural features in healthy breast tissue to robustly estimate risk up to five years after screening. This texture model generalized across mammographic devices from different vendors.
The AI system, the texture model, and other patient characteristics were all aggregated in a combination model resulting in a rich mammography-based risk profile that advanced state-of-the-art breast cancer risk assessment.
The models of this thesis could support clinicians in tailoring personalized screening strategies which in turn will benefit patients and radiologists.
Supervisors
Principal Supervisor Martin Lillholm
Clinical co-supervisor Ilse Vejborg
Assessment Committee
Professor Erik Bjørnager Dam, Department of Computer Science (Leader of defence)
Professor Sebastién Ourselin, King's College London
Professor Carla Henrica van Gils, Julius Center (JC) for Health Sciences and Primary Care, University Medical Center Utrecht
For an electronic copy of the thesis, please visit the PhD Programme page.