PhD defence by Ola Rønning
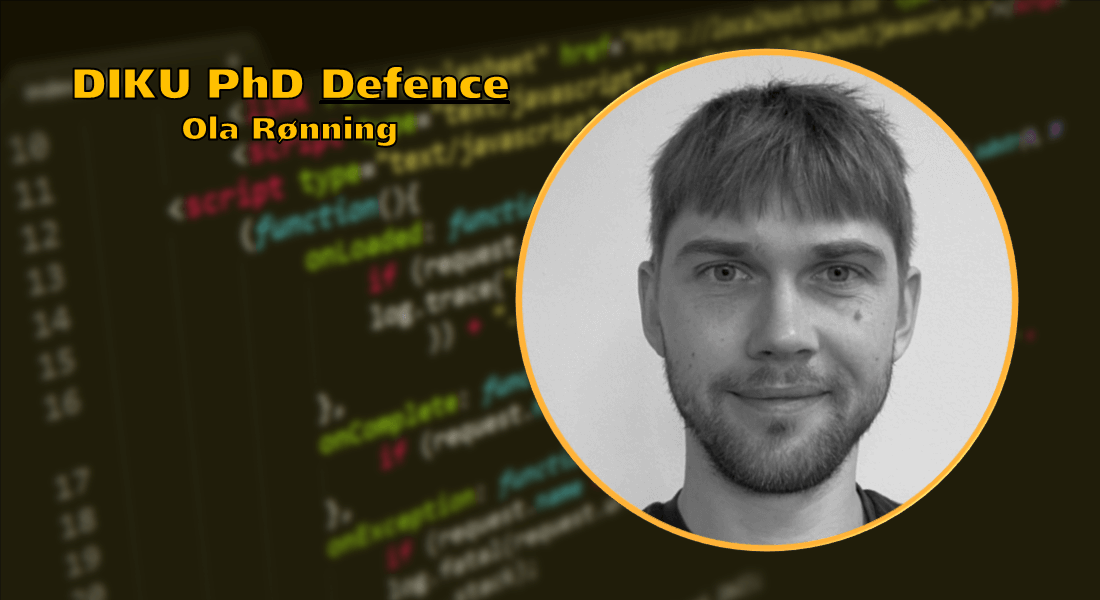
Title
A Probabilistic Approach to the Protein Folding Problem
Abstract
In the first part of this thesis, we extend Stein mixtures (a Stein variational gradient descent variate) to a whole class of approximate inference algorithms indexed by a scalar. We recommend the best choice of indexing scalar and demonstrate why by analyzing the gradient noise. We also present a ready-to-use library for inference with Stein mixtures as an extension to the NumPyro probabilistic programming language (PPL). The library, called EinStein, includes the black box Stein mixture inference engine, automatic guide generation, many studied kernels, and copiable examples of Bayesian neural networks and deep Markov models.
In the second part of the thesis, we study the protein structure prediction problem as a showcase for applying PPLs in the natural sciences. The protein prediction problem aims to predict the (ensemble of) conformation(s) a particular protein may adopt(s) given its sequence of amino acids (and potentially known protein homologs). A high-fidelity solution to the problem could have a massive impact on treatment for misfolding diseases such as cancer, Alzheimer's, Huntington's, and Parkinson's. A canonical representation of a protein conformation is its internal (toroidal) coordinates. Internal coordinates allow efficient updates to the protein's three-dimensional structure without violating physiochemical properties. To infer statistical models over internal coordinate representations, we introduce a variate of the bivariate von Mises distribution (a 2-torus distribution) in the (Num)Pyro \gls{PPL}s. The distribution (known as the sine distribution) enables us to specify a hierarchical model over the two high-variance backbone torsion angles. Our model captures probable angle pairs for each amino acid order of magnitude faster than preexisting methods.
Finally, we present our preliminary results on inferring a distribution over protein folding forcefields. Current technologies for protein structure prediction are excellent at the single-structure forecast. However, these methods are black box deep models and yield no insights into physiochemical properties--sometimes even violating them. Our formulation of the folding force as a probabilistic program allows us to automate the tedious process of tuning protein folding forcefields using our Stein mixture inference engine.
Supervisors
Principal Supervisor Thomas Wim Hamelryck
Co-supervisor Christophe Ley, University of Luxemburg
Assessment Committee
Professor Yevgeny Seldin, DIKU
Professor Søren Hauberg, DTU
Principal scientist Martin Jankowiak, Generate Biomedicines, Cambridge, MA, USA
Leader of defense: Yevgeny Seldin
For an electronic copy of the thesis, please visit the PhD Programme page.