PhD defence by Per Steffen Czolbe
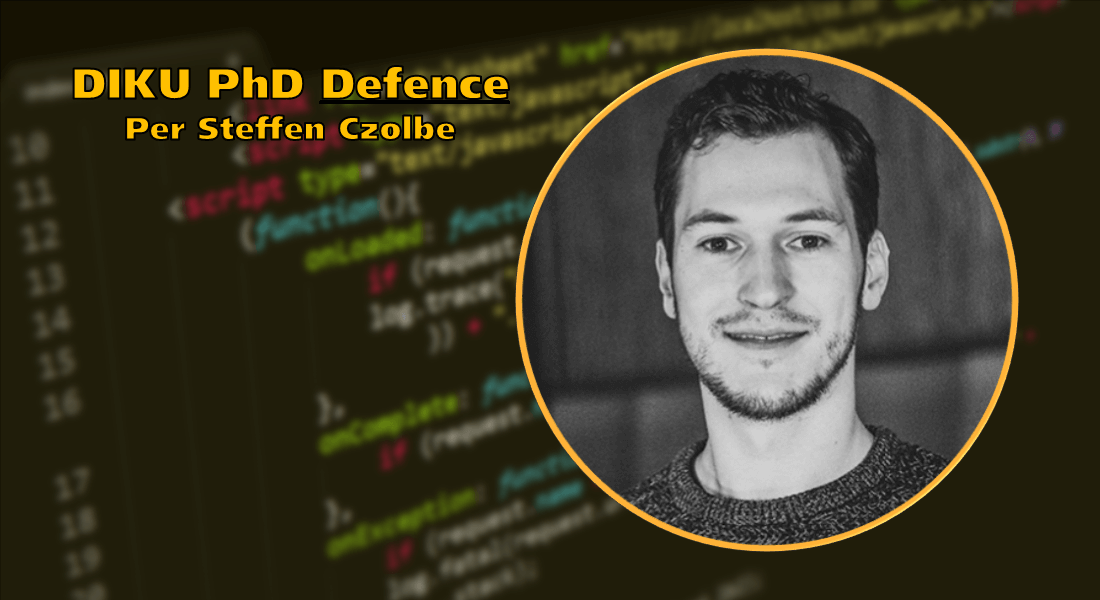
Title
Topology-Aware Image Registration
Abstract
Deformable image registration, or the nonlinear alignment of images, is a fundamental preprocessing tool in medical imaging. Existing registration methods regularize this ill-posed problem by the assumption of a common topology across all images. Images are assumed to be one-to-one deformations of a common reference template. However, this assumption is frequently violated in the real world, especially among populations requiring medical intervention, where the physical anatomy can differ from a common template due to tumors or surgical resections. In such cases, the same-image assumption is often accepted as unavoidable, leading to imprecise registration and inaccuracies in the subsequent analysis. This is especially troubling as medical imaging tools are most often used to process the non-standard anatomies of patients requiring treatment, not healthy reference populations.
Over the last few years, deep learning methods have enabled fundamentally new approaches to image registration. Unsupervised learning-based registration models have achieved performance on par with classical algorithms while being many times faster. However, current deep-learning-based registration is still based on the one-to-one matching of images, thus suffering from the same shortcomings under a change of anatomy.
This thesis explores new opportunities enabled by deep learning to overcome the same-image assumption, such as implicitly inferring a solution from observed data with an unsupervised probabilistic approach and informing the optimization with semantic image representations. However, adopting deep learning to medical imaging also introduces new challenges. The thesis contributes to the open research questions of selecting a suitable model architecture for image registration, quantifying model uncertainty in the presence of annotator variability, and training few-shot models in a clinical research environment.
These strands of work combine into an unsupervised model for detecting anatomical differences alongside the registration step. The model highlights areas where the registration is inadequate due to violations of the same-image assumption, indicating where further care has to be taken in the analysis and downstream processing.
This is an important milestone toward a fully topology-aware image registration and an essential building block toward a deformable registration pipeline for images with differences in anatomy.
Supervisors
Principal Supervisor Christian Igel
Former Principal Supervisor Aasa Feragen
Co-supervisor Oswin Krause
Assessment Committee
Associate Professor Melanie Ganz-Benjaminsen, Computer Science
Professor Enzo Ferrante, Universidad Torcuato Di Tella and Universidad de San Andrés
Associate Professor Islem Rekik, Imperial College London
Leader of defence: Melanie Ganz-Benjaminsen
For an electronic copy of the thesis, please visit the PhD Programme page.