DIKU Bits: Measuring fairness in recommender systems: can we trust the results?
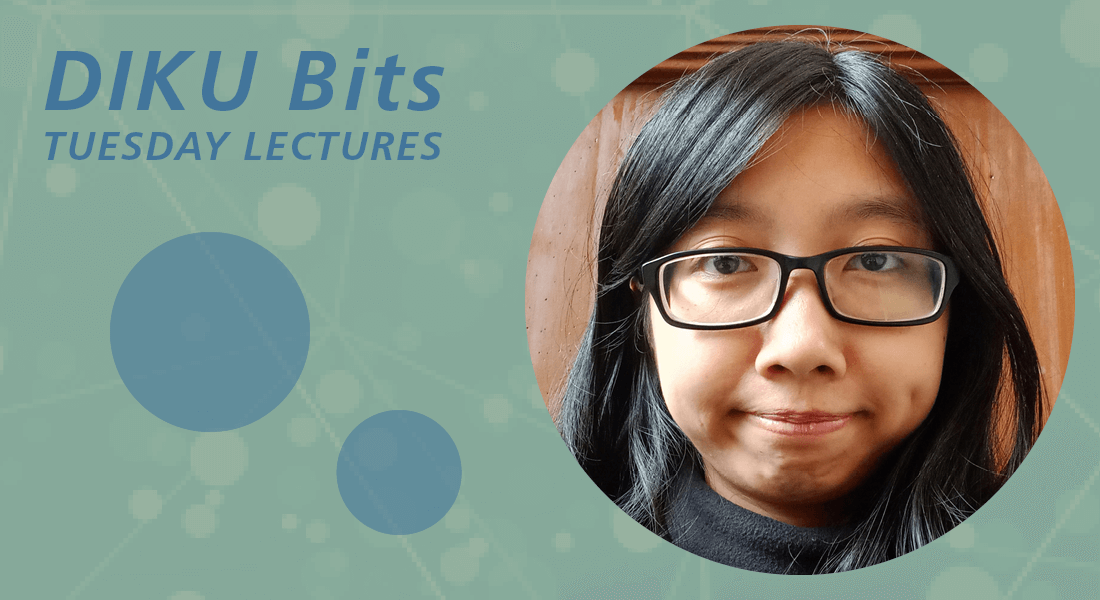
Title
Measuring fairness in recommender systems: can we trust the results?
Speaker
Theresia Veronika Rampisela, PhD student in the Machine Learning (ML) section
Abstract
From social media to job platforms, recommender systems are inseparable from our lives. Now, the goal of these systems has evolved beyond accuracy: the recommended contents should also be fair. What kind of recommendations is fair? Fair for whom? How do we measure fairness? This talk introduces fairness evaluation in recommender systems, focusing on why achieving a perfectly (un)fair score is theoretically impossible, how fairness scores can be deceiving, and the solutions to these limitations.
Which courses do you teach? (BSc and MSc)
I am a teaching assistant (TA) for Web Recommender Systems (WRS).
Which technology/research/projects/startup are you excited to see the evolution of?
Anything related to Trustworthy and Responsible AI, especially how they are evaluated in practice!