PhD defence by Lei Li
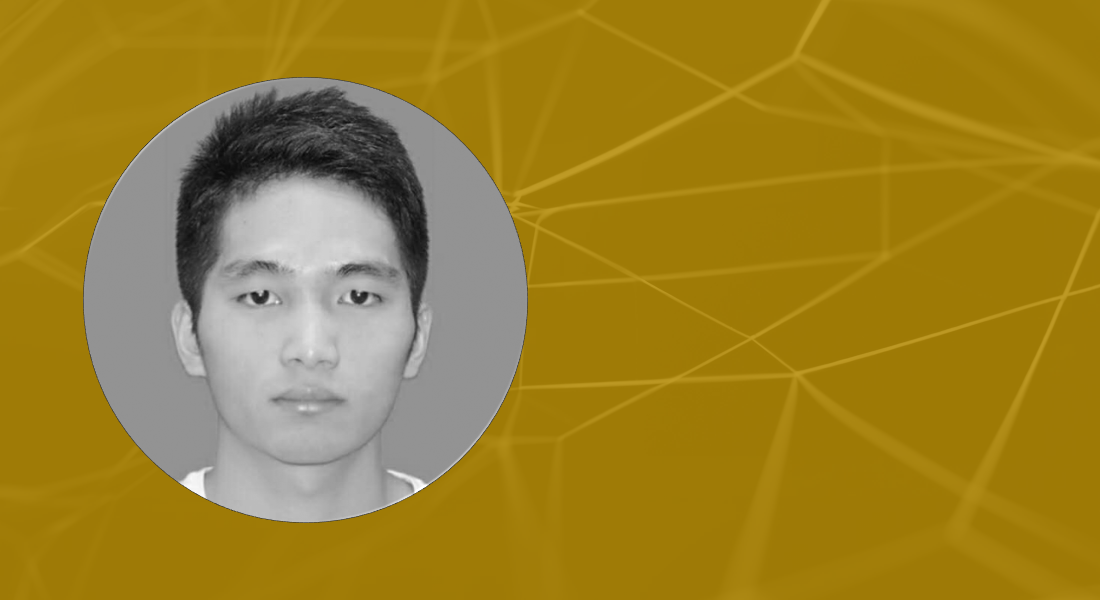
Follow this link to participate on Zoom
Title
Contributions to deep learning for computer vision applied to environmental remote sensing and human face and pose analysis
Abstract
This thesis presents research in deep learning for computer vision with applications to remote sensing data and human face and pose analysis. The focus is on 3D data, and various input modalities are considered: images, 3D point clouds, and natural language.
The first part of the thesis considers remote sensing of the environment. The first contribution in this domain is the use of Chain-of-Thought language prompting to enhance semantic image segmentation accuracy, particularly in challenging scenarios like flood disasters. This approach fuses visual and linguistic elements.
The second study considers aligning and fusing diverse modalities for the segmentation of buildings in satellite imagery.
The third study considers the prediction of aboveground forest biomass based on 3D point clouds from airborne LiDAR, for example for measuring carbon sequestration. We suggest replacing the current analysis of handcrafted statistical features derived from the point clouds by applying point cloud neural networks for regression directly to the 3D data. Then edgeaware learning for 3D point clouds is proposed, which addresses the challenges of noise in point cloud data by focusing on edge features to improve classification and segmentation.
The second part of the thesis focuses on the analysis of data from humans. The first work presented involves systems capable of real-time face segmentation, which includes accurate face detection, alignment, and parsing. These systems leverage 3D facial features and can handle occlusions and diverse facial expressions. The final study addresses human pose estimation, which finds extensive application in fields such as augmented reality/virtual reality (AR/VR), live broadcasting, and interactive media. It enhances the user experience by providing more realistic and responsive interactions. The proposed method leverages zero-shot learning algorithms to accurately capture and analyze human movements and poses with diffusion generation methods in uncontrolled environments.
We propose various methods for a range of applied computer vision tasks, utilizing different data modalities. Our research encompasses several scenarios, unified by a core challenge: effectively employing deep learning networks for the analysis of varied modalities.
Supervisors
Principal Supervisor Christian Igel
Assessment Committee
Professor Kim Steenstrup Pedersen, Computer Science
Professor Yifang Ban, KTH, Sweden
Professor Daniel Sonntag, Oldenburg University, Germany
For an electronic copy of the thesis, please visit the PhD Programme page.