PhD defence by Sebastián Garcia Lopez
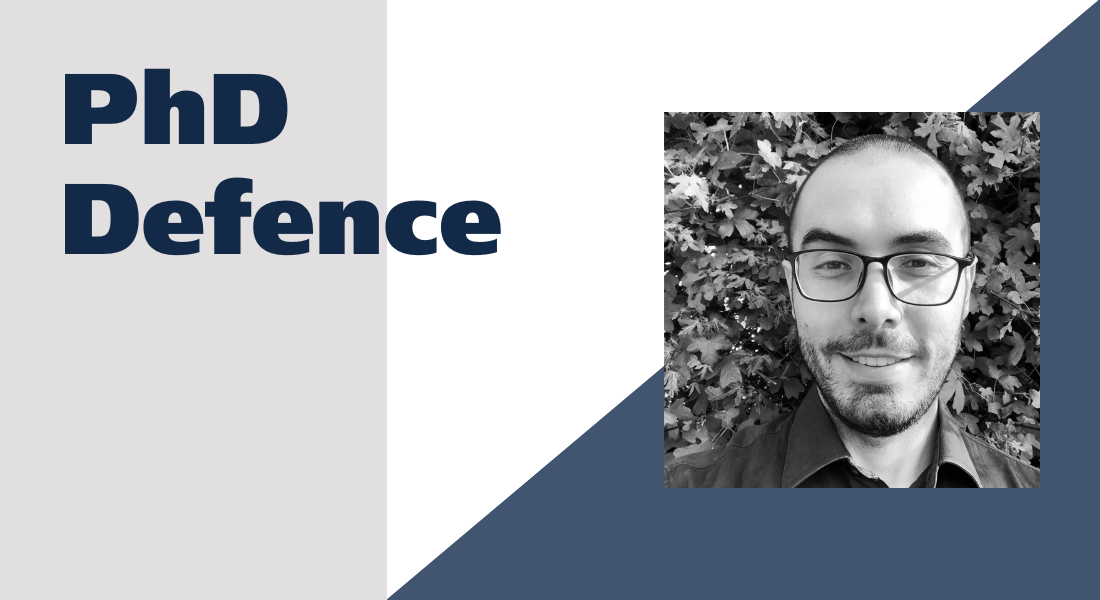
Title
Representation Learning in Protein Science: From Sequence Alignments to Deep Embedding Spaces
Abstract
In the field of protein modelling, protein engineering and bioinformatics in general, two main approaches to protein representation have emerged as the spearheads for a wide range of applications, particularly in machine learning tasks: representations based on Multiple Sequence Alignment (MSA) features and the representation based on embedding spaces. MSA-based representations have long been the gold standard in the field and continue to play an important role, even contributing to the development of algorithms such as AlphaFold2, underlining their continued relevance. However, the rise of embedding spaces has gained tremendous momentum with the advent of Protein Language Models (pLMs), which have become central to many state-of-the-art protein representation algorithms without the need for alignments, such as ESM2 and ProtBERT. Nevertheless, there is no clear guideline or path on when to favour one approach over the other, as both are highly relevant and offer distinct advantages depending on the task, leaving room for further exploration and research in this area.
This thesis aims to offer two contributions to the scientific community concerning these two types of representations. The first contribution is algorithmic, where we propose, as a proof-of-concept, a novel strategy for MSA based on deep generative models and spatial transformations. In this initial contribution, we frame MSA as a spatial transformation problem, providing robust and generalizable alignments for new sequences through the creation of a probabilistic graphical model based on ensembles of variational encoders. The second contribution addresses the prediction of a widely used proxy for protein thermostability: melting temperatures through embedding-based representations. While many state-of-the-art methods in this area depend on global metrics to evaluate model performance, these can often obscure important issues, such as the significant inter-species imbalance within the datasets. This work addresses this challenge and proposes strategies for effectively inducing regression models in which the imbalance between species is prominent.
Supervisors
Principal Supervisor Wouter Krogh Boomsma
Assessment Committee
Professor Anders Krogh, Computer Science
Professor Wim Vranken, Artificial Intelligence Lab, Vrije Universiteit Brussel
Professor Jes Frellsen, DTU Compute, DTU
For an electronic copy of the thesis, please visit the PhD Programme page.