PhD defence by Simone Borg Bruun
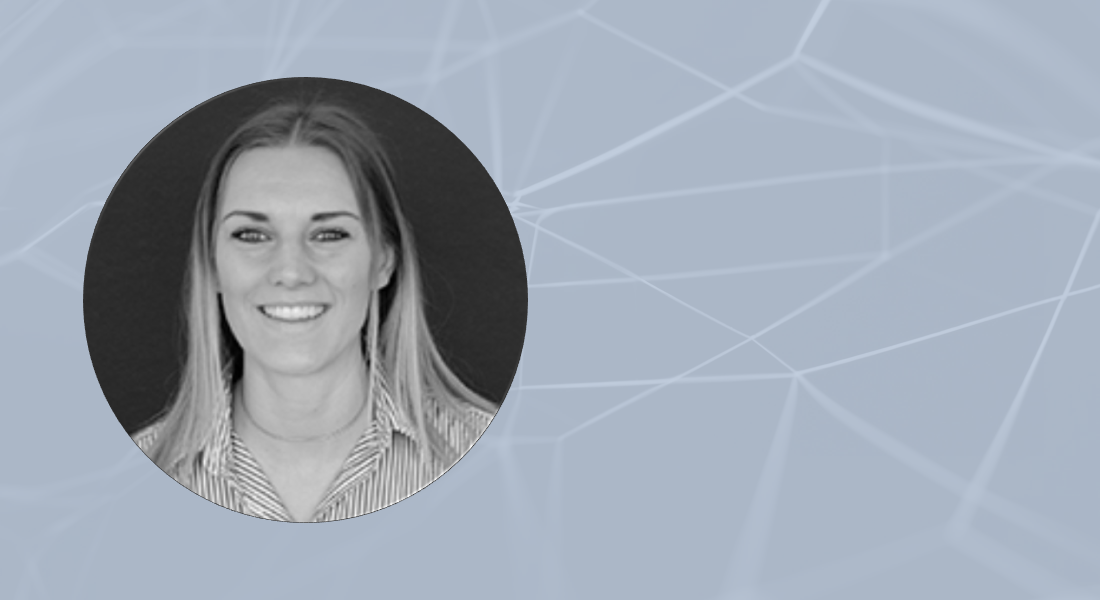
Title
Learning Recommendations from Sparse and Heterogeneous Data
Abstract
Recommender systems have gained great success in helping users to navigate online services with a large variety of items, such as movies, music, retail and news, by inferring the users’ preferences from their feedback on items like ratings, views and purchases. Personalized recommendations are also highly relevant in domains with few items, like the insurance domain, to guide the users’ decision-making of products that are complex because they have many components and complex pricing. The process of generating recommendations in such domains raises some serious challenges with sparse and heterogeneous data that are under-researched in the field of recommender systems.
Thus, this thesis studies the generation of personalized recommendations under the special characteristics of complex item domains. Existing methods do not apply to complex item domains, because of the following reasons. The methods rely on a large volume of user feedback on items (e.g., purchases), but in complex item domains, the user feedback is sparse. This is because there may be few different items, and the items are not interacted with very often. Moreover, existing methods infer users’ preferences for movie actors, music genres and so forth, whereas users have needs rather than preferences for complex products that tend to be temporal as their lives change. Finally, in complex item domains, the user feedback is of multiple types and modalities, such as actions on the website and phone calls with service agents, that are challenging to handle for existing methods. This is because they assume all feedback to be interactions with items (e.g., ratings or purchases) and they can, for example, not deal with a modality being missing. In addition to generating recommendations, we further study the process of generating explanations of recommendations under the special characteristics of complex item domains. Explanations of recommendations try to address the problem of explaining why items are recommended to a user. It is required by law and relevant for many domains, including complex item domains, to increase the trust and transparency of complex product recommendations.
In summary, this thesis contributes improved recommendation and explanation methods that are suited for the aforementioned challenges within complex item domains, with a particular focus on tailor-made solutions for sparse-data recommendations, temporal needs and heterogeneous feedback in recommender systems. Collectively these contributions advance the state-of-the-art in recommender systems for this particular domain in ways that are scientifically interesting, timely, and societally relevant.
Supervisors
Principal Supervisor Christina Amalia Lioma, DIKU
Co-Supervisor Maria Maistro, DIKU
Assessment Committee
Professor Panagiotis Karras, DIKU
Professor Min Zhang, Tsinghua University
Professor Olfa Nasraoui, University of Louisville
For an electronic copy of the thesis, please visit the PhD Programme page.