PhD defence by Yijie Zhang
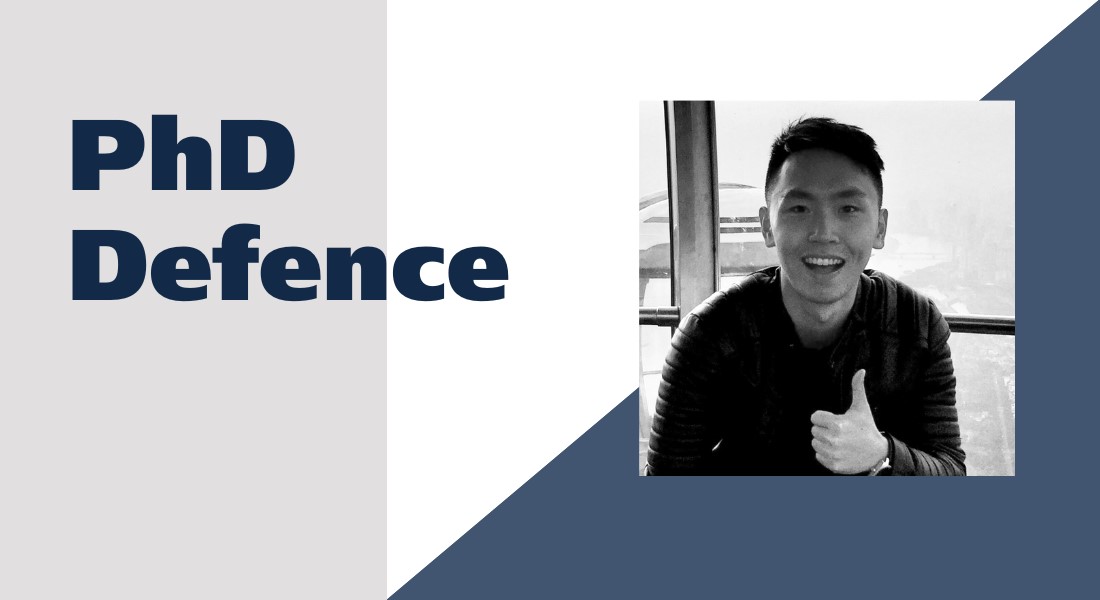
Title
On Cold Posteriors of Probabilistic Neural Networks: Understanding the Cold Posterior Effect and A New Way to Learn Cold Posteriors with Tight Generalization Guarantees
Abstract
Bayesian inference provides a principled probabilistic framework for quantifying uncertainty by updating beliefs based on prior knowledge and observed data through Bayes’ theorem. In Bayesian deep learning, neural network weights are treated as random variables with prior distributions, allowing for a probabilistic interpretation and quantification of predictive uncertainty. However, Bayesian methods lack theoretical generalization guarantees for unseen data. PAC-Bayesian analysis addresses this limitation by offering a frequentist framework to derive generalization bounds for randomized predictors, thereby certifying the reliability of Bayesian methods in machine learning.
Temperature T, or inverse-temperature λ =1/T, originally from statistical mechanics in physics, naturally arises in various areas of statistical inference, including Bayesian inference and PAC-Bayesian analysis. In Bayesian inference, when T < 1 (“cold” posteriors), the likelihood is up-weighted, resulting in a sharper posterior distribution. Conversely, when T > 1 (“warm” posteriors), the likelihood is down-weighted, leading to a more diffuse posterior distribution. By balancing the influence of observed data and prior regularization, temperature adjustments can address issues of underfitting or overfitting in Bayesian models, bringing improved predictive performance.
We begin by investigating the cold posterior effect (CPE) in Bayesian deep learning. We demonstrate that misspecification leads to CPE only when the Bayesian posterior underfits. Additionally, we show that tempered posteriors are valid Bayesian posteriors corresponding to different combinations of likelihoods and priors parameterized by temperature T. Fine-tuning T thus allows for the selection of alternative Bayesian posteriors with less misspecified likelihood and prior distributions.
Next, we introduce an effective PAC-Bayesian procedure, Recursive PAC-Bayes (RPB), that enables sequential posterior updates without information loss. This method is based on a novel decomposition of the expected loss of randomized classifiers, which reinterprets the posterior loss as an excess loss relative to a scaled-down prior loss, with the latter being recursively bounded. We show empirically that RPB significantly outperforms prior works and achieves the best generalization guarantees.
We then explore the connections between Recursive PAC-Bayes, cold posteriors (T < 1), and KL-annealing (where T increases from 0 to 1 during optimization), showing how RPB’s update rules align with these practical techniques and providing new insights into RPB’s effectiveness.
Finally, we present a novel evidence lower bound (ELBO) decomposition for mean-field variational global latent variable models, which could enable finer control of the temperature T. This decomposition could be valuable for future research, such as understanding the training dynamics of probabilistic neural networks.
Supervisors
Principal Supervisor Christian Igel
Co-Supervisor Merete Bang
Co-Supervisor Sadegh Talebi
Assessment Committee
Associate Professor Oswin Krause, Computer Science
Professor Asja Fischer, Ruhr-University Bochum, Germany
Associate Professor Jes Frellsen, Technical University of Denmark
Leader of defense: Oswin Krause
IT responsible person: Lasse Kristensen
For an electronic copy of the thesis, please visit the PhD Programme page.