Fighting Harmful Content on the Web
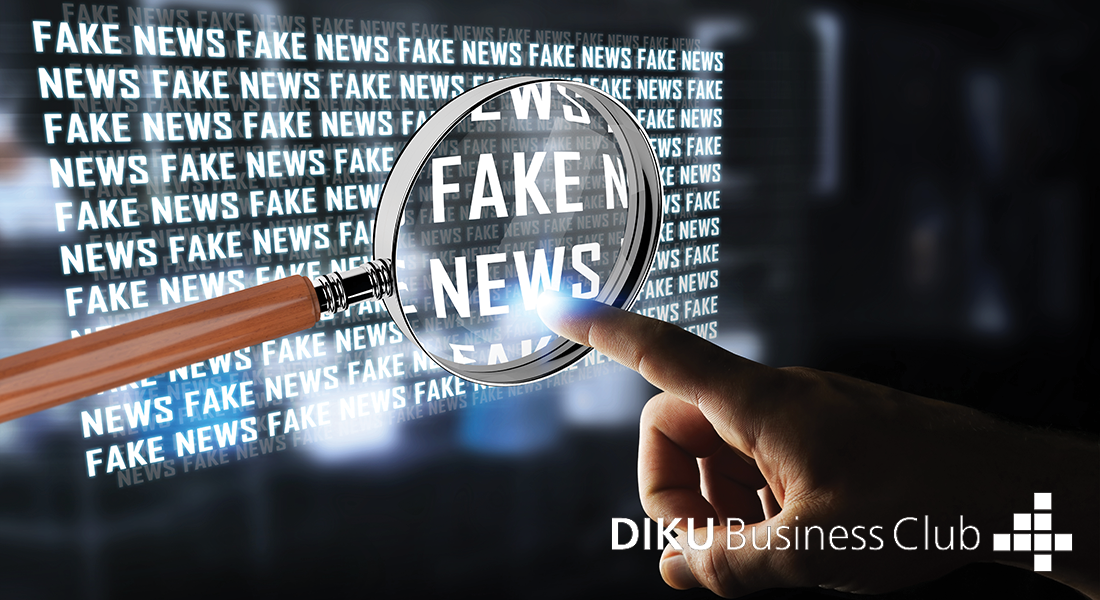
Fake news, hate speech and biased search algorithms have become some of the biggest problems in today's media landscape. How can AI methods be used to combat these problems? Are the solutions to these problems democratic or do they raise the barrier for traditional media entrants? Are we heading towards a streaming future and what challenges does this present for content moderation workflows?
In this webinar, you can learn how researchers and industry practitioners collaborate on strategies to reduce harmful content on the Web — including what some of the solutions are - and how it can help decision-makers, including governmental bodies and social media platform operators.
Who should participate?
If you are interested in fake news detection and the technology behind NLP, Natural Language Processing, an upcoming field of research related to Artificial Intelligence and Machine Learning, you should join this webinar, whether as a researcher, a media creator, a decision-maker or simply in your capacity of a media user.
The webinar is open to all interested persons, but you need to register to follow the webinar in Zoom.
Programme
14.00 | Welcome | Anders Pall Skött, Head of Business and Innovation, DIKU |
14.05 | Towards Explainable Fact-Checking | Isabelle Augenstein, Associate Professor, DIKU |
14.30 | Misinformation and Search Engines | Maria Maistro, Assistant Professor, DIKU |
14.50 | Short break | |
15.00 | Content Moderation - A Strategic Priority for Leaders by 2025 | Jonathan Manfield, CTO, CheckStep |
15.20 | Q&A Session | Moderated by Anders Pall Skött |
Presentations and speakers
Brief welcome session by Anders Pall Skött, Head of Business and Innovation at DIKU, who will introduce today's theme and speakers.
Abstract:
The past decade has seen a substantial rise in the amount of mis- and disinformation online, from targeted disinformation campaigns to influence politics, to the unintentional spreading of misinformation about public health. This development has spurred research in the area of automatic fact checking, from approaches to detect check-worthy claims and determining the stance of tweets towards claims, to methods to determine the veracity of claims given evidence documents.
These automatic methods are often content-based, using natural language processing methods, which in turn utilise deep neural networks to learn higher-order features from text in order to make predictions. As deep neural networks are black-box models, their inner workings cannot be easily explained. At the same time, it is desirable to explain how they arrive at certain decisions, especially if they are to be used for decision making. While this has been known for some time, the issues this raises have been exacerbated by models increasing in size, and by EU legislation requiring models to be used for decision making to provide explanations, and, very recently, by legislation requiring online platforms operating in the EU to provide transparent reporting on their services. Despite this, current solutions for explainability are still lacking in the area of fact checking.
A further general requirement of such deep learning based method is that they require large amounts of in-domain training data to produce reliable explanations. As automatic fact checking is a very recently introduced research area, there are few sufficiently large datasets. As such, research on how to learn from limited amounts of training data, such as how to adapt to unseen domains, is needed.
This talk provides a brief introduction to the area of automatic fact checking, including claim check-worthiness detection, stance detection and veracity prediction. It then presents some first solutions on generating explanations for fact checking.
Bio
Isabelle Augenstein is Associate Professor and heads the The NLP Research Section at DIKU.
Abstract:
The spread of fake news and misinformation has become a severe issue affecting society in many ways. For example, it can influence the public opinion to promote a political candidate and substantially affect the final election outcome, or it can have deleterious effects on people reputation, especially on social networks. When it comes to health, it can harm people by guiding them to take wrong decisions, which, in the worst cases, can lead people to injure themselves. During the COVID-19 pandemic, we all witness how misinformation can be dangerous and have severe consequences on people choices and life.
In this context, search engines are in a central position, as they can play an active role in contrasting the spread of misinformation. Web pages that are relevant, credible and incorrect represent a serious threat for users, and should be discarded or presented very low in the ranking. There is a call to design search engines that can promote relevant, credible and correct information over incorrect information.
This talk will present an overview of ongoing research and solutions in the context of fake news and search engines, as well as some preliminary results.
Bio:
Maria Maistro studied initially Mathematics and then Computer Science (PhD, University of Padua, 2018). She is a tenure track assistant professor at the Department of Computer Science, University of Copenhagen (DIKU). Prior to this, she was a postdoctoral researcher at the Department of Computer Science, University of Copenhagen (DIKU) and at the University of Padua in Italy. She conducts research in information retrieval, and particularly on evaluation, reproducibility and replicability, click log analysis, expert search, learning to rank and applied machine learning. She has already co-organized several international scientific events and she has served as member of programme committees and reviewer for highly ranked conferences and journals in information retrieval.
Abstract:
In the wake of a generational pandemic, social media is rife with deadly misinformation and geopolitical tensions are further stretched by peak racial sentiment. We are more prepared to fight fire and theft than we are to defend against destructive threats to modern enterprise and humanity. Set against the backdrop of prophesied growth of a new generation of social networks (Social+) and a dynamic legislative landscape, we explore the tools in the toolbox for oversight boards and platform integrity teams to grow healthy online communities.
Through the intersectional lens of business, technology and ethics, we paint the picture of the true costs of operating a service with a social element and outline the AI we are developing at CheckStep to provide organisations with compliant-first ability to uphold terms of service.
We discuss:
- Statement of reason, the clause in new legislation that could be the forcing function for XAI
- Throughout 2020, dubious claims about elections and COVID-19 have increased pressure on government and platforms to act, events reached a climax during the crisis at the capitol and provoked an unprecedented response. What is the role of reporting in a deplatforming decision?
- Are we heading to a streaming future and what challenges does this present for content moderation workflows? Are solutions to these problems democratic or do they raise the barrier for traditional media entrants?
- Recent developments in NLP are an enabler for the technology we wish to develop - but can AI also be tech for bad? - Can generative technologies such as Deepfake and GPT-3 be wielded by malicious actors to flood defense systems?
Bio:
Jonathan Manfield is CTO at CheckStep. Engineering Lead from FinTech. Has Developed mission-critical systems for real-time risk management and payments. AI Executive Diploma cohort member at Saïd Business School (Oxford).
Ask questions to the panellists - moderated by Anders Pall Skött.